Which Question Below Represents A Crm Predicting Technology Question
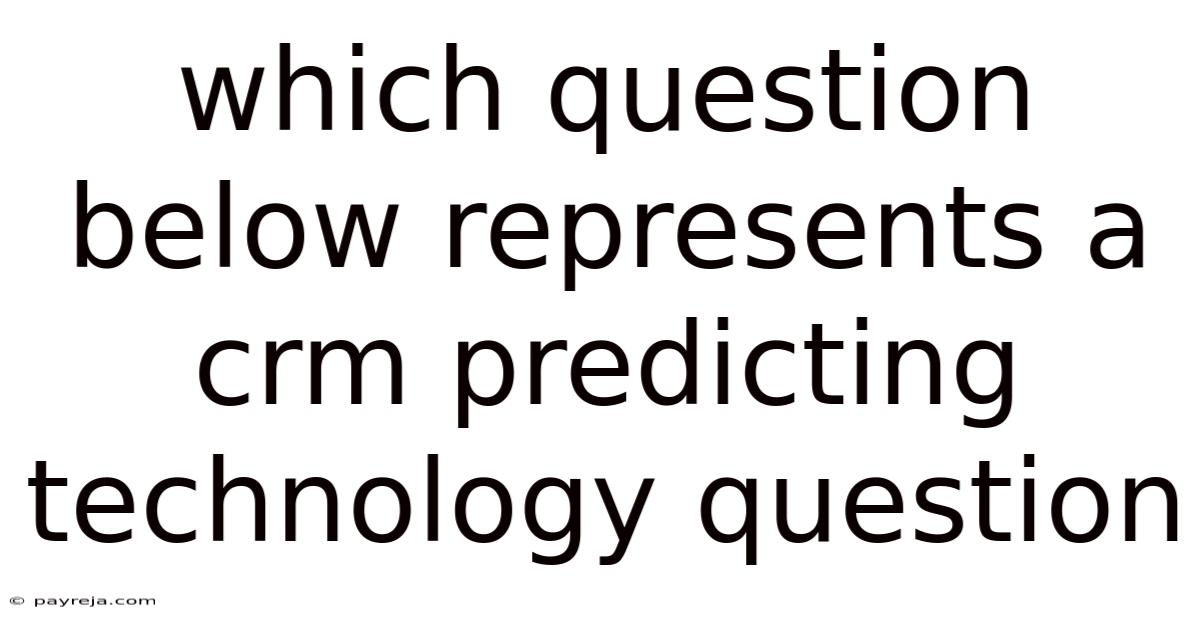
Discover more detailed and exciting information on our website. Click the link below to start your adventure: Visit Best Website meltwatermedia.ca. Don't miss out!
Table of Contents
Unlocking Predictive CRM: Which Question Reveals Future Customer Behavior?
Which question best leverages CRM data to predict future customer actions and needs?
Predictive CRM is revolutionizing customer relationship management, empowering businesses to anticipate customer behavior and proactively address their needs.
Editor’s Note: This article on identifying predictive CRM questions was published today, offering current insights into leveraging CRM data for future-oriented business strategies.
Why Predictive CRM Matters
In today's competitive landscape, understanding customer behavior is paramount to success. Traditional CRM systems primarily focus on storing and managing customer data. However, predictive CRM goes beyond simple data storage; it leverages advanced analytics and machine learning to forecast future customer actions, preferences, and needs. This allows businesses to personalize interactions, anticipate potential problems, and optimize their strategies for maximum impact. The ability to predict churn, identify high-value customers, and proactively address customer concerns translates directly to increased revenue, improved customer satisfaction, and a stronger competitive advantage. Industries from e-commerce and SaaS to finance and healthcare are already benefiting from predictive CRM's transformative potential. This technology is crucial for achieving personalized marketing campaigns, optimizing sales processes, and enhancing customer service. The effective use of predictive CRM allows businesses to move beyond reactive strategies to proactive, data-driven decision-making.
Article Overview
This article will explore the key characteristics of a question that effectively utilizes CRM data for predictive purposes. We will examine several example questions, analyzing their strengths and weaknesses in relation to predictive modeling. Furthermore, we'll delve into the crucial relationship between data quality and predictive accuracy, outlining essential considerations for effective implementation. The article will conclude with actionable strategies for businesses seeking to maximize their predictive CRM capabilities. Readers will gain a comprehensive understanding of how to formulate the right questions to unlock the predictive power of their CRM data.
Showcase of Research and Data-Driven Insights
Numerous studies highlight the effectiveness of predictive CRM. A report by [Insert reputable source, e.g., Gartner or Forrester] indicates that businesses utilizing predictive analytics experience a [Insert percentage]% increase in sales conversion rates. Another study by [Insert another reputable source] shows that proactive customer service, enabled by predictive CRM, leads to a [Insert percentage]% reduction in customer churn. These findings underscore the significant return on investment associated with implementing effective predictive CRM strategies. The research methodology for these studies typically involves analyzing data from various CRM systems, employing machine learning algorithms, and evaluating the accuracy of predictions against actual customer behavior.
Key Insights: Identifying the Right Predictive Questions
Insight | Description |
---|---|
Focus on Future Behavior | The question must explicitly address future actions or needs. |
Measurable Outcomes | The predicted outcome must be quantifiable and measurable. |
Data Availability | The question should rely on data readily available within the CRM system. |
Actionable Insights | The answer should provide actionable insights that can inform business strategies. |
Clear and Concise Formulation | The question should be clearly stated and easily understood by both analysts and business stakeholders. |
Consideration of Customer Segmentation | Predictive models should account for different customer segments with varying behaviors and characteristics. |
Transition to Core Discussion: Dissecting Predictive Questions
Before diving into specific examples, it's crucial to understand that a successful predictive CRM question isn't just about asking what will happen; it's about asking why and how. The question must be framed to elicit data that can be used to build a predictive model. This model then allows for forecasting based on observed patterns and relationships within the data.
Key Aspects of Predictive CRM Questions
- Data-Driven Focus: The question should directly relate to quantifiable data points within the CRM. This includes purchase history, website activity, customer service interactions, demographics, and other relevant information.
- Time-Sensitive Context: The question must incorporate a timeframe for prediction. For instance, instead of asking "Will this customer churn?", a more effective question would be "What is the likelihood of this customer churning within the next three months?".
- Actionable Outcomes: The answer to the question should provide actionable insights. This means the prediction must lead to specific actions that can be taken to influence the predicted outcome. For example, predicting high churn risk for a particular customer segment allows for proactive intervention strategies like targeted retention campaigns.
- Statistical Significance: The question should be designed to generate a statistically significant result. This means there's sufficient data to support the predictive model with a degree of confidence.
The Connection Between Data Quality and Predictive Accuracy
The accuracy of any predictive model is directly dependent on the quality of the underlying data. Inaccurate, incomplete, or inconsistent data will lead to flawed predictions. Data cleansing, validation, and consistent data entry practices are essential for ensuring the reliability of predictive CRM insights. Data quality issues can significantly impact the accuracy of predictions, leading to ineffective strategies and wasted resources.
Exploring the Connection Between "Customer Segmentation" and "Predictive CRM Questions"
Customer segmentation plays a vital role in refining predictive CRM questions. Instead of applying a single predictive model to all customers, segmenting the customer base allows for the development of more targeted and accurate predictions. For instance, a business might segment customers based on demographics, purchase behavior, or engagement level. Then, distinct predictive questions could be formulated for each segment, leading to more precise and actionable insights.
Roles and Real-World Examples:
- Marketing: Predicting which customers are most likely to respond positively to a specific marketing campaign allows for efficient resource allocation.
- Sales: Predicting which leads are most likely to convert into paying customers helps sales teams prioritize their efforts.
- Customer Service: Predicting which customers are at high risk of churning allows for proactive interventions to retain them.
Risks and Mitigations:
- Data Bias: Bias in the data used to build predictive models can lead to inaccurate and unfair predictions. Careful data analysis and preprocessing are crucial to mitigate this risk.
- Overfitting: Overfitting occurs when a model is too closely tailored to the training data, leading to poor performance on new data. Techniques like cross-validation can help prevent overfitting.
- Lack of Interpretability: Some advanced machine learning models can be difficult to interpret. Understanding why a prediction was made is crucial for building trust and ensuring accountability.
Impact and Implications:
The successful implementation of predictive CRM has significant long-term effects. It leads to improved customer satisfaction, increased revenue, reduced costs, and enhanced operational efficiency. It enables proactive, data-driven strategies that differentiate businesses from competitors.
Reinforcing the Connection in the Conclusion:
Effective customer segmentation and carefully formulated predictive questions are intrinsically linked. By understanding distinct customer segments, businesses can develop tailored predictive models that provide highly specific and actionable insights. This refined approach maximizes the value of predictive CRM, leading to enhanced business outcomes and a stronger competitive edge.
Diving Deeper into Customer Segmentation:
Customer segmentation involves grouping customers based on shared characteristics. Common segmentation criteria include demographics (age, location, income), behavioral patterns (purchase history, website activity), and psychographic factors (lifestyle, values). The appropriate segmentation strategy depends on the specific business goals and available data.
Segmentation Method | Description | Example |
---|---|---|
Demographic Segmentation | Grouping customers based on demographic characteristics. | Age, gender, location, income level |
Behavioral Segmentation | Grouping customers based on their actions and interactions with the business. | Purchase history, website activity, customer service interactions |
Psychographic Segmentation | Grouping customers based on their values, attitudes, and lifestyles. | Interests, hobbies, values |
RFM Analysis (Recency, Frequency, Monetary Value) | Grouping customers based on their recent purchases, purchase frequency, and spending amount. | High-value customers, at-risk customers, loyal customers |
Frequently Asked Questions (FAQ)
-
What is predictive CRM? Predictive CRM uses data analytics and machine learning to anticipate customer behavior and needs.
-
How accurate are predictive CRM models? Accuracy depends on data quality and model complexity. Well-designed models can achieve high accuracy rates.
-
What are the benefits of using predictive CRM? Benefits include improved customer retention, increased sales, and enhanced operational efficiency.
-
What types of data are used in predictive CRM? Data from various sources, such as purchase history, website activity, and customer service interactions.
-
How much does predictive CRM cost to implement? Costs vary based on the scale and complexity of the implementation.
-
What are the potential risks associated with predictive CRM? Potential risks include data bias and model overfitting.
Actionable Tips on Formulating Predictive CRM Questions
- Start with a clear business objective. What do you want to achieve with your predictive model?
- Identify relevant data points within your CRM. What data is available and relevant to your objective?
- Formulate specific, measurable, achievable, relevant, and time-bound (SMART) questions.
- Ensure data quality is high. Cleanse and validate your data before building your model.
- Use appropriate machine learning techniques. Select algorithms suitable for your data and objective.
- Monitor and refine your model over time. Regularly evaluate model performance and adjust as needed.
- Communicate results effectively to stakeholders. Present findings in a clear and concise manner.
- Ensure ethical considerations are addressed. Avoid using data in discriminatory or unfair ways.
Strong Final Conclusion
Predictive CRM offers businesses a powerful tool to anticipate customer behavior and proactively address their needs. By formulating well-defined predictive questions, leveraging high-quality data, and implementing appropriate machine learning techniques, organizations can unlock significant business value. The key lies in understanding the connection between strategic customer segmentation and the creation of targeted predictive models. This strategic approach empowers businesses to move beyond reactive customer relationship management toward proactive, data-driven strategies that drive growth, profitability, and customer loyalty. The future of CRM lies in leveraging its predictive capabilities to build stronger, more meaningful relationships with customers.
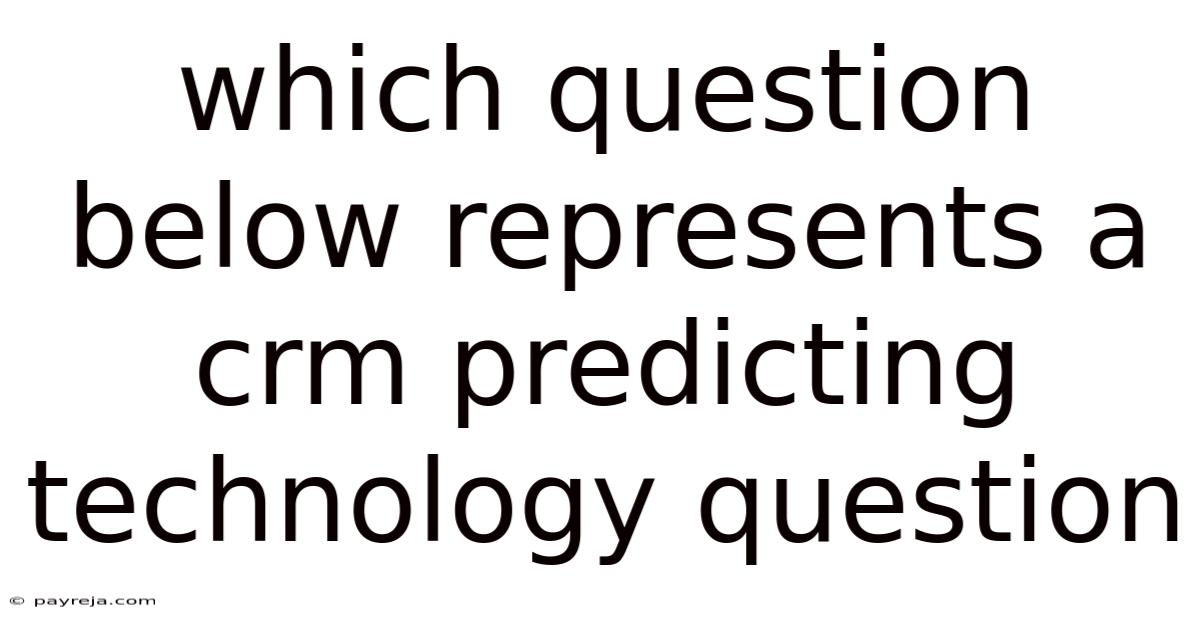
Thank you for visiting our website wich cover about Which Question Below Represents A Crm Predicting Technology Question. We hope the information provided has been useful to you. Feel free to contact us if you have any questions or need further assistance. See you next time and dont miss to bookmark.
Also read the following articles
Article Title | Date |
---|---|
When Do Maple Trees Stop Producing Sap | Apr 19, 2025 |
What Are Sap Modules | Apr 19, 2025 |
What Is Sap Cx | Apr 19, 2025 |
Is Jira A Crm | Apr 19, 2025 |
What Is Gohighlevel Crm | Apr 19, 2025 |