What Is A Key Advanced Technology Behind Crm Analytics
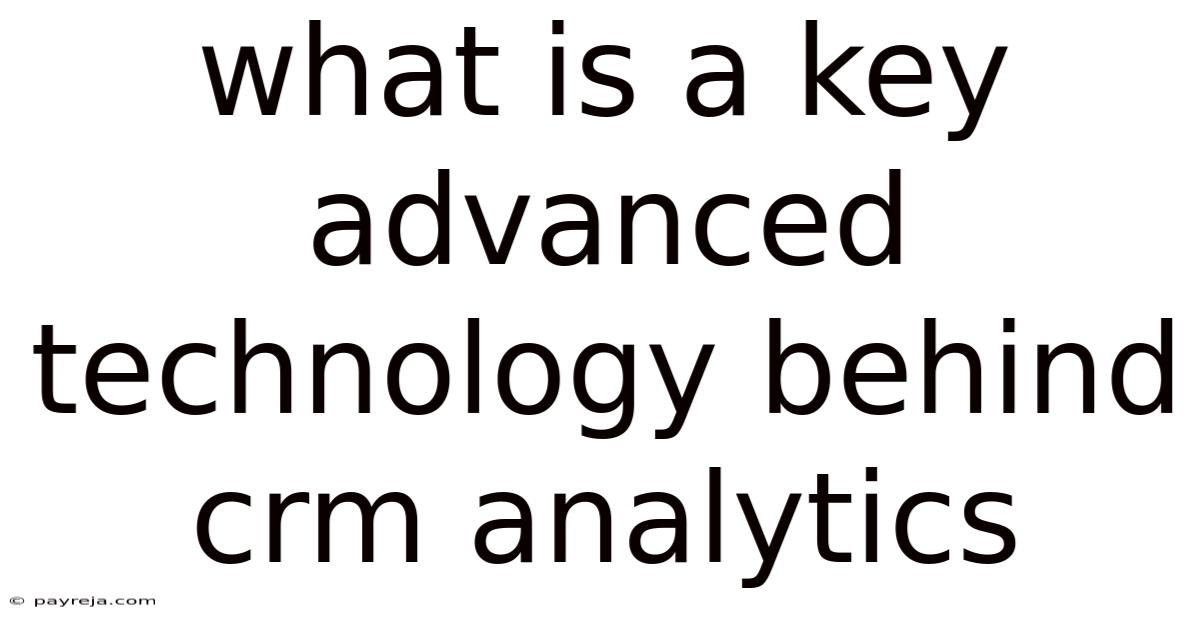
Discover more detailed and exciting information on our website. Click the link below to start your adventure: Visit Best Website meltwatermedia.ca. Don't miss out!
Table of Contents
Unlocking CRM Analytics: The Power of Predictive Modeling
What if unlocking the secrets of predictive modeling could revolutionize your CRM strategy? This advanced technology is transforming how businesses understand customer behavior and drive revenue growth.
Editor’s Note: This article on predictive modeling in CRM analytics has been updated today to reflect the latest advancements and industry best practices.
Predictive modeling is arguably the most impactful advanced technology driving the evolution of CRM analytics. It moves beyond simply reporting on past performance to anticipate future customer behavior, enabling businesses to make proactive, data-driven decisions. This capability empowers organizations to personalize customer interactions, optimize marketing campaigns, improve sales forecasting, and ultimately boost profitability. Understanding its intricacies is crucial for any business aiming to leverage the full potential of its CRM system.
This article will explore the core principles of predictive modeling within the context of CRM analytics. It will delve into its various applications, the key factors influencing its effectiveness, and the practical steps businesses can take to implement and optimize this transformative technology. Readers will gain a comprehensive understanding of predictive modeling's capabilities and its impact on modern CRM strategies.
What is Predictive Modeling in CRM?
Predictive modeling leverages historical data from a CRM system to build statistical models that forecast future outcomes. Instead of simply describing what happened, it predicts what will happen, offering valuable insights into customer churn risk, sales opportunities, and marketing campaign effectiveness. This process involves using various statistical algorithms and machine learning techniques to identify patterns and relationships within the data, ultimately generating probability scores or predictions for specific events.
These predictions aren't just educated guesses; they’re based on rigorous analysis of factors such as customer demographics, purchase history, website activity, support interactions, and social media engagement. By combining these disparate data points, predictive models create a holistic view of each customer, enabling more targeted and effective interventions.
Key Applications of Predictive Modeling in CRM
The applications of predictive modeling in CRM are vast and constantly evolving. Some key areas where it delivers significant value include:
-
Customer Churn Prediction: Identifying customers at high risk of churning allows businesses to proactively intervene with targeted retention strategies, such as personalized offers or improved customer service.
-
Sales Opportunity Scoring: Predictive models can assess the likelihood of closing a sales deal based on various factors, allowing sales teams to prioritize high-potential leads and allocate resources effectively.
-
Marketing Campaign Optimization: By predicting which customers are most likely to respond positively to specific marketing messages, businesses can personalize campaigns and maximize their ROI. This includes identifying optimal channels, messaging, and timing for different customer segments.
-
Product Recommendation: Analyzing customer purchase history and browsing behavior enables the recommendation of relevant products, increasing sales and improving customer satisfaction.
-
Cross-selling and Upselling: Predicting which customers are most likely to purchase additional products or services allows for targeted cross-selling and upselling opportunities.
-
Pricing Optimization: Predictive modeling can help determine optimal pricing strategies based on customer segmentation, market conditions, and competitor analysis.
Data Sources and Model Building
Effective predictive modeling relies on high-quality data from various sources integrated within the CRM system. This includes:
-
CRM Data: This forms the backbone of predictive modeling, encompassing customer demographics, purchase history, interactions with support teams, and marketing campaign engagement.
-
External Data: Integrating external data sources such as social media activity, website analytics, and economic indicators can significantly enrich the models and improve prediction accuracy.
The model-building process typically involves the following steps:
-
Data Cleaning and Preparation: This crucial step involves handling missing values, correcting inconsistencies, and transforming data into a suitable format for analysis.
-
Feature Engineering: Selecting and transforming relevant variables (features) that best predict the desired outcome.
-
Model Selection: Choosing an appropriate statistical or machine learning algorithm based on the data and the prediction task (e.g., logistic regression, decision trees, random forests, neural networks).
-
Model Training and Evaluation: Training the chosen model on historical data and evaluating its performance using appropriate metrics (e.g., accuracy, precision, recall, AUC).
-
Model Deployment and Monitoring: Deploying the trained model into the CRM system and continuously monitoring its performance to ensure accuracy and adapt to changing patterns.
The Interplay Between Predictive Modeling and CRM Functionality
Predictive modeling isn't a standalone technology; it's deeply integrated with various CRM functionalities. For instance, customer segmentation features within the CRM system can be significantly enhanced by incorporating predictive models to create more accurate and relevant segments. Similarly, marketing automation tools can be leveraged to deliver personalized messages and offers based on the predictions generated by the model. The sales force automation module can benefit from predictive scoring of leads, helping prioritize interactions and improve sales conversion rates. Reporting and analytics dashboards are transformed by the inclusion of predictive insights, enabling a more proactive and data-driven approach to decision-making.
Key Factors Influencing Predictive Model Accuracy
Several factors influence the accuracy and effectiveness of predictive models in CRM:
-
Data Quality: High-quality, comprehensive data is essential. Inaccurate, incomplete, or inconsistent data can lead to flawed predictions.
-
Model Complexity: Overly complex models can overfit the training data, resulting in poor generalization to new, unseen data. A balance between model complexity and predictive power is crucial.
-
Feature Selection: Selecting the right features is crucial. Including irrelevant or redundant features can reduce model accuracy and increase computational costs.
-
Algorithm Selection: The choice of algorithm significantly influences model performance. Different algorithms perform best under different circumstances.
-
Regular Monitoring and Updates: Models need to be regularly monitored and updated to reflect changes in customer behavior and market conditions.
Risks and Mitigation Strategies
While predictive modeling offers significant advantages, businesses need to be aware of potential risks:
-
Bias in Data: If the training data reflects existing biases, the model may perpetuate and even amplify these biases, leading to unfair or discriminatory outcomes. Careful attention to data preprocessing and model validation is crucial to mitigate this risk.
-
Data Privacy Concerns: Using customer data for predictive modeling raises privacy concerns. Businesses must comply with all relevant data protection regulations and ensure data security.
-
Overreliance on Predictions: While predictive models provide valuable insights, they should not be the sole basis for decision-making. Human judgment and experience remain vital.
-
Lack of Explainability: Some complex models, such as deep neural networks, can be "black boxes," making it difficult to understand how they arrive at their predictions. This lack of explainability can hinder trust and adoption.
Case Studies: Real-World Applications
Numerous businesses have successfully implemented predictive modeling in their CRM systems, achieving significant improvements in key metrics. For example, a telecommunications company used predictive modeling to identify customers at high risk of churning. By proactively offering targeted retention deals, they significantly reduced churn rates and improved customer lifetime value. Similarly, a retail company employed predictive modeling to personalize product recommendations, leading to a substantial increase in sales and improved customer engagement. These examples demonstrate the transformative power of predictive modeling in driving business growth.
Actionable Tips for Implementing Predictive Modeling in CRM
-
Start Small: Begin by focusing on a specific use case, such as churn prediction or sales opportunity scoring.
-
Invest in Data Quality: Ensure your CRM data is accurate, complete, and consistent.
-
Collaborate with Experts: Partner with data scientists or analytics consultants to build and deploy effective models.
-
Monitor and Evaluate: Regularly monitor model performance and make necessary adjustments.
-
Focus on Explainability: Prioritize models that offer insights into their predictions.
-
Embrace Continuous Improvement: Predictive modeling is an iterative process. Continuously refine your models based on feedback and new data.
Frequently Asked Questions
Q1: What type of CRM is best suited for predictive modeling?
A1: Any CRM system that allows for robust data integration and customization can be used for predictive modeling. However, cloud-based CRMs often provide more flexibility and scalability for handling large datasets and integrating with external data sources.
Q2: How much does predictive modeling cost?
A2: The cost varies depending on the complexity of the model, the data sources used, and the level of expertise required. It can range from a relatively low cost for simple models to a significant investment for complex, custom-built solutions.
Q3: How long does it take to implement predictive modeling?
A3: The implementation time depends on various factors, including data preparation, model building, and integration with the CRM system. It can range from a few weeks to several months.
Q4: What are the ethical considerations of using predictive modeling in CRM?
A4: Ethical considerations include ensuring fairness, transparency, and data privacy. Businesses must comply with all relevant regulations and avoid perpetuating biases in their models.
Q5: What are the key performance indicators (KPIs) for evaluating predictive models?
A5: KPIs depend on the specific application but might include accuracy, precision, recall, AUC (Area Under the Curve), lift, and ROI.
Q6: Can small businesses implement predictive modeling?
A6: Yes, even small businesses can benefit from predictive modeling. There are various affordable tools and services available, and starting with a limited scope can make implementation manageable.
Conclusion
Predictive modeling is a powerful technology that is transforming CRM analytics. By enabling businesses to anticipate customer behavior and make proactive decisions, it delivers significant value across various departments. While implementing predictive modeling requires careful planning and execution, the potential benefits—improved customer retention, increased sales, optimized marketing campaigns, and ultimately, greater profitability—make it a worthwhile investment for businesses of all sizes seeking a competitive edge in today's data-driven world. The future of CRM is undeniably intertwined with the continued advancement and broader adoption of predictive modeling.
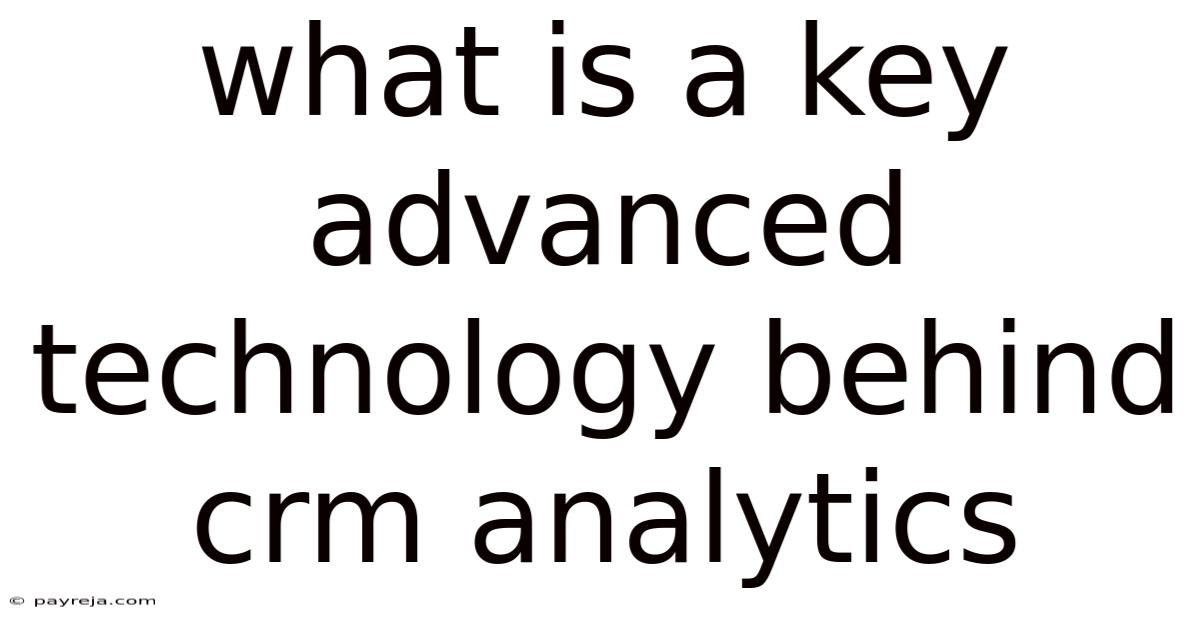
Thank you for visiting our website wich cover about What Is A Key Advanced Technology Behind Crm Analytics. We hope the information provided has been useful to you. Feel free to contact us if you have any questions or need further assistance. See you next time and dont miss to bookmark.
Also read the following articles
Article Title | Date |
---|---|
Tutorial Teachmint | Apr 19, 2025 |
What Is Sap Sales And Distribution | Apr 19, 2025 |
How Long Can You Store Maple Sap | Apr 19, 2025 |
Is Gorgias A Crm System | Apr 19, 2025 |
What Crm Specialist Do | Apr 19, 2025 |