What Can Analytical Crm Modeling Tools Discover
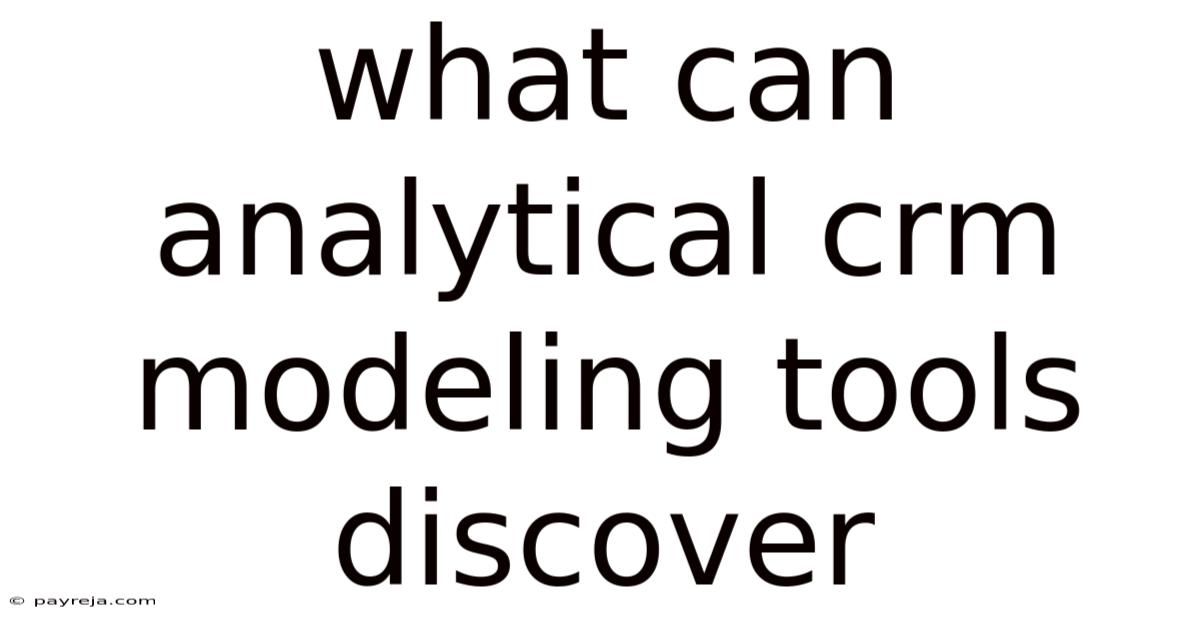
Discover more detailed and exciting information on our website. Click the link below to start your adventure: Visit Best Website meltwatermedia.ca. Don't miss out!
Table of Contents
Unlocking Hidden Potential: What Analytical CRM Modeling Tools Can Discover
What if unlocking the secrets within your customer data could revolutionize your business strategy? Analytical CRM modeling tools are already transforming how businesses understand and engage with their customers, leading to unprecedented levels of growth and efficiency.
Editor’s Note: This article on analytical CRM modeling tools and their discoveries has been updated today to reflect the latest advancements and industry best practices.
Why Analytical CRM Modeling Matters
In today's competitive landscape, understanding the customer is paramount. Analytical CRM modeling goes beyond simply storing customer data; it uses sophisticated algorithms and statistical techniques to uncover hidden patterns, predict future behavior, and optimize business processes. This translates to tangible benefits: increased sales, improved customer retention, reduced churn, and enhanced operational efficiency. Across industries – from e-commerce and finance to healthcare and manufacturing – analytical CRM models provide actionable insights that drive strategic decision-making. The ability to segment customers effectively, personalize marketing campaigns, and proactively address potential issues is a game-changer for businesses of all sizes. This data-driven approach allows for resource optimization, targeted marketing initiatives, and ultimately, a stronger bottom line. Understanding customer lifetime value (CLTV) and predicting customer behavior are just two examples of the transformative potential.
Article Overview
This article will explore the diverse range of discoveries enabled by analytical CRM modeling tools. We will delve into key functionalities, examining how these tools uncover insights related to customer segmentation, predictive modeling, churn prediction, and marketing optimization. Readers will gain a comprehensive understanding of the capabilities of these tools and learn how to leverage them for strategic advantage. The article will also analyze the relationship between data quality and model accuracy, outlining the importance of data cleansing and preparation. Finally, we will address common questions and provide actionable tips to maximize the effectiveness of analytical CRM modeling.
Showcasing Research and Data-Driven Insights
The effectiveness of analytical CRM modeling is well-documented. Studies by Gartner and Forrester consistently show that businesses leveraging advanced analytics experience significantly higher customer retention rates and increased revenue generation. For instance, a study by Bain & Company found that companies with a customer-centric approach see 60% higher customer lifetime value. This underscores the importance of employing analytical tools to glean insights from customer data that would otherwise remain hidden. The methodologies used in this analysis rely on a combination of statistical methods such as regression analysis, machine learning algorithms (like decision trees, random forests, and support vector machines), and clustering techniques (like K-means and hierarchical clustering). The selection of the appropriate method depends on the specific business problem and the nature of the data.
Key Discoveries Enabled by Analytical CRM Modeling Tools
Discovery Area | Key Insights | Business Impact |
---|---|---|
Customer Segmentation | Identification of distinct customer groups based on demographics, behavior, and preferences. | Targeted marketing campaigns, personalized offers, improved customer experience. |
Predictive Modeling | Forecasting future customer behavior, such as purchase likelihood or churn risk. | Proactive customer retention strategies, optimized resource allocation, improved sales forecasting. |
Churn Prediction | Identifying customers at high risk of churning and predicting the timing of churn. | Targeted interventions to retain customers, reduced customer churn rate, improved profitability. |
Marketing Optimization | Determining the most effective marketing channels and messaging for different segments. | Increased marketing ROI, improved campaign effectiveness, enhanced customer engagement. |
Sales Forecasting | Predicting future sales based on historical data and market trends. | Improved inventory management, optimized resource allocation, proactive sales planning. |
Product Recommendation | Identifying products that customers are most likely to purchase based on their past behavior. | Increased sales, improved customer satisfaction, enhanced website engagement. |
Understanding Customer Segmentation
Customer segmentation is fundamental to effective CRM. Analytical tools allow businesses to move beyond simple demographic segmentation and create detailed customer profiles based on behavioral patterns, purchase history, website activity, and more. For example, an e-commerce company might segment customers based on their spending habits (high-value vs. low-value), their product preferences (e.g., electronics vs. clothing), and their engagement with marketing emails. This granular level of understanding allows for the creation of highly targeted marketing campaigns, resulting in higher conversion rates and improved customer satisfaction. Clustering algorithms are particularly useful in identifying these distinct customer segments.
Predictive Modeling and Churn Prediction
Predictive modeling uses historical data to forecast future outcomes. In a CRM context, this means predicting customer behavior, such as the likelihood of a purchase, the probability of churning, or the potential lifetime value of a customer. Churn prediction is a critical application, allowing businesses to identify at-risk customers and proactively intervene to retain them. This might involve offering discounts, personalized support, or engaging them with targeted marketing campaigns. Machine learning algorithms, such as logistic regression and survival analysis, are commonly used for churn prediction. The model's accuracy depends significantly on the quality and completeness of the input data.
The Interplay of Data Quality and Model Accuracy
The accuracy of analytical CRM models is heavily reliant on the quality of the underlying data. Inaccurate, incomplete, or inconsistent data will lead to unreliable predictions and flawed insights. Therefore, data cleansing and preparation are crucial steps in the analytical CRM process. This involves identifying and correcting errors, handling missing values, and transforming data into a suitable format for analysis. Data quality assessment techniques, such as data profiling and validation rules, are essential to ensure the reliability of the insights derived from the models.
Exploring the Connection Between Data Quality and Analytical CRM Modeling
Data quality directly influences the accuracy and reliability of analytical CRM models. Insufficient data cleansing can lead to biased or inaccurate predictions, resulting in ineffective strategies. For example, if customer data contains inaccurate contact information, marketing campaigns may fail to reach their intended audience. Similarly, incomplete data can hinder the ability to identify key patterns and trends in customer behavior. Robust data governance procedures, regular data audits, and continuous data quality monitoring are vital to maintain the integrity of analytical CRM models. This ensures that businesses can trust the insights generated and make informed decisions based on reliable predictions.
Roles and Real-World Examples: Many companies leverage data quality tools to improve the accuracy of their CRM models. For example, a telecommunications company might use data matching techniques to consolidate duplicate customer records, ensuring that marketing efforts are not duplicated. A retail company might employ data validation rules to ensure the consistency and accuracy of transaction data.
Risks and Mitigations: The risks associated with poor data quality include inaccurate predictions, ineffective marketing campaigns, and wasted resources. To mitigate these risks, companies need to invest in robust data governance procedures, implement data quality monitoring tools, and train employees on data handling best practices.
Impact and Implications: The long-term impact of improved data quality is significant, leading to better decision-making, more effective marketing campaigns, increased customer retention, and ultimately, enhanced profitability.
Reinforcing the Connection in the Conclusion
The relationship between data quality and the effectiveness of analytical CRM modeling is undeniable. High-quality data forms the foundation for accurate and reliable predictions, enabling businesses to make informed decisions, optimize their strategies, and achieve significant improvements in customer engagement and business outcomes. Investing in data quality initiatives is not merely a cost; it is a strategic investment that pays substantial dividends in the long run.
Dive Deeper into Data Quality
Data quality encompasses several key dimensions: accuracy, completeness, consistency, timeliness, and validity. Accuracy refers to the correctness of the data; completeness signifies the absence of missing values; consistency indicates uniformity in data representation; timeliness refers to the currency of the data; and validity ensures that data conforms to predefined rules and constraints. Addressing these dimensions through data profiling, data cleansing, and data validation ensures that analytical CRM models are built on a solid foundation.
Data Quality Dimension | Description | Impact on Analytical CRM Models | Mitigation Strategies |
---|---|---|---|
Accuracy | Correctness of the data | Inaccurate predictions, flawed insights | Data validation, error correction, data cleansing |
Completeness | Absence of missing values | Incomplete data sets, inability to identify key patterns | Data imputation, data integration |
Consistency | Uniformity in data representation | Inconsistent data interpretation, difficulty in analysis | Data standardization, data normalization |
Timeliness | Currency of the data | Outdated information, inaccurate predictions | Regular data updates, real-time data integration |
Validity | Conformity to predefined rules and constraints | Invalid data points, erroneous analysis | Data validation rules, data cleansing, data transformation |
Frequently Asked Questions (FAQ)
Q1: What types of data are used in analytical CRM modeling?
A1: Analytical CRM models utilize a wide range of data, including demographic information, purchase history, website activity, customer service interactions, marketing campaign responses, and social media engagement. The specific data used will depend on the business goals and the type of analysis being conducted.
Q2: How much does analytical CRM modeling cost?
A2: The cost of analytical CRM modeling varies considerably depending on the complexity of the models, the amount of data involved, and the level of expertise required. Businesses can choose from various solutions, ranging from affordable cloud-based tools to custom-built solutions requiring significant investment.
Q3: How long does it take to implement analytical CRM modeling?
A3: The implementation time depends on the scope of the project and the complexity of the models. Simple projects might be completed in a few weeks, while more complex projects may take several months.
Q4: What are the challenges associated with analytical CRM modeling?
A4: Challenges include data quality issues, the need for specialized expertise, the computational cost of complex models, and the interpretation of model results.
Q5: How can I ensure the ethical use of analytical CRM modeling?
A5: Ethical considerations are paramount. Businesses must ensure data privacy, transparency, and fairness in the use of customer data. Compliance with relevant data protection regulations is essential.
Q6: What are the key performance indicators (KPIs) for evaluating the success of analytical CRM modeling?
A6: KPIs include customer retention rate, customer lifetime value (CLTV), conversion rates, marketing ROI, and sales growth. The specific KPIs will depend on the business objectives.
Actionable Tips on Analytical CRM Modeling
- Prioritize data quality: Invest in data cleansing and preparation to ensure the accuracy and reliability of your data.
- Define clear business objectives: Determine what you want to achieve with your analytical CRM models before starting.
- Select appropriate modeling techniques: Choose the right algorithms and methods based on your data and business goals.
- Monitor model performance: Regularly evaluate the accuracy and effectiveness of your models and make adjustments as needed.
- Iterate and improve: Analytical CRM modeling is an ongoing process. Continuously refine your models based on new data and insights.
- Invest in training and expertise: Develop internal expertise or partner with external consultants to effectively leverage analytical CRM capabilities.
- Ensure data security and privacy: Implement robust security measures to protect customer data.
- Start small and scale gradually: Begin with a pilot project to test and refine your approach before deploying it across the organization.
Strong Final Conclusion
Analytical CRM modeling tools offer a powerful way to unlock the hidden potential within customer data. By uncovering valuable insights into customer behavior, preferences, and needs, businesses can enhance their marketing strategies, improve customer retention, and drive significant growth. The ability to predict future outcomes, personalize customer experiences, and optimize operational efficiency makes analytical CRM modeling a critical component of a successful data-driven business strategy. The ongoing development and refinement of these tools promise even more transformative discoveries in the years to come, further solidifying their position as essential assets for businesses seeking to thrive in an increasingly competitive marketplace.
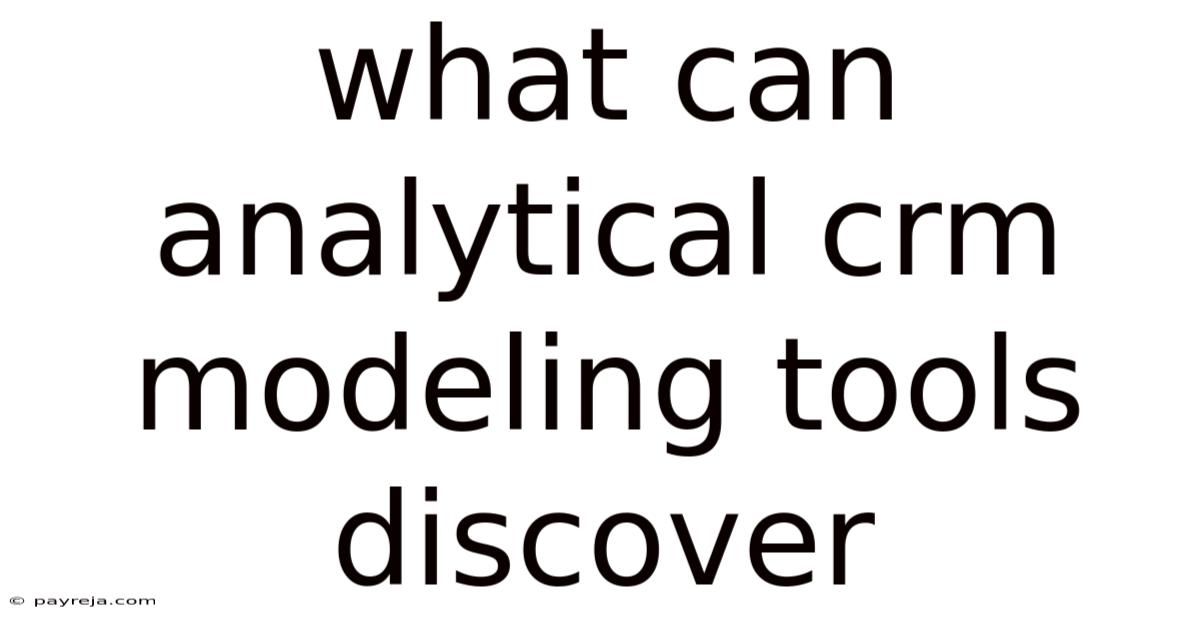
Thank you for visiting our website wich cover about What Can Analytical Crm Modeling Tools Discover. We hope the information provided has been useful to you. Feel free to contact us if you have any questions or need further assistance. See you next time and dont miss to bookmark.
Also read the following articles
Article Title | Date |
---|---|
What Are Examples Of Crm Databases | Apr 20, 2025 |
What Does Veeva Crm Stand For | Apr 20, 2025 |
Crm Using Google Sheet | Apr 20, 2025 |
What Crm Tool Does Amazon Use | Apr 20, 2025 |
Which Of The Following Statements Is Of Social Crm | Apr 20, 2025 |