Visual Matrix
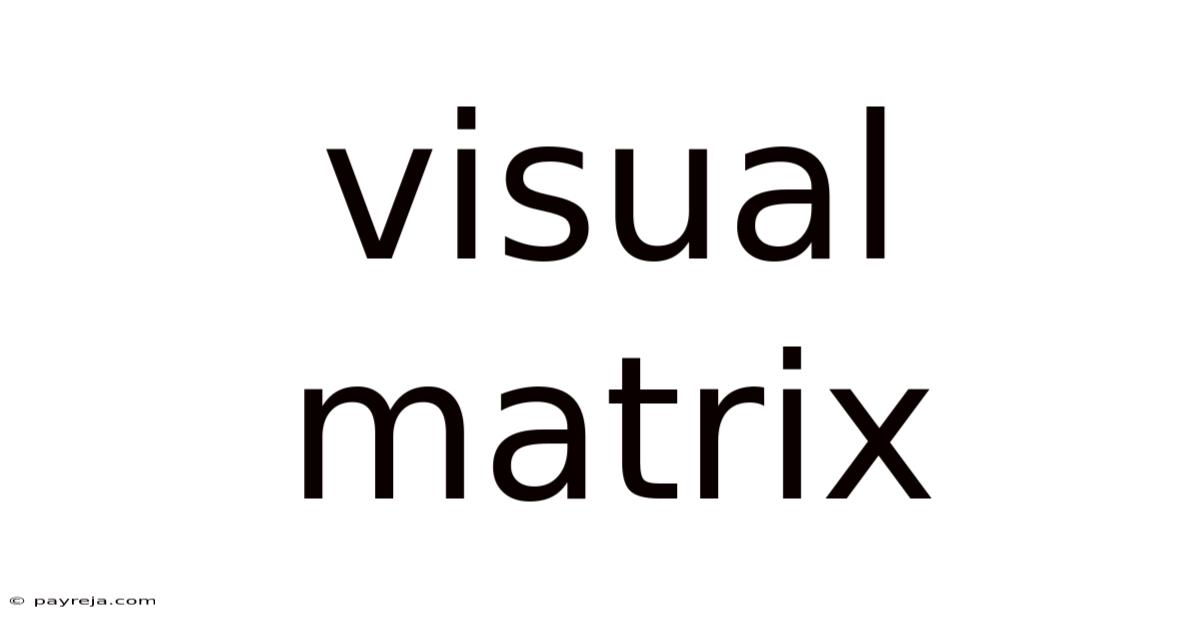
Discover more detailed and exciting information on our website. Click the link below to start your adventure: Visit Best Website meltwatermedia.ca. Don't miss out!
Table of Contents
Unveiling the Power of Visual Matrices: Insights and Applications
What if harnessing the power of visual matrices could revolutionize how we process information and solve complex problems?
Visual matrices are rapidly transforming how data is visualized and interpreted across diverse fields.
Editor’s Note: This article on visual matrices has been updated today to reflect the latest advancements and applications in this rapidly evolving field.
Visual matrices, at their core, are visual representations of data arranged in rows and columns. This seemingly simple structure unlocks a powerful ability to identify patterns, relationships, and insights that might otherwise remain hidden within raw data. Their impact spans various sectors, from business intelligence and market research to scientific data analysis and project management. The ability to quickly grasp complex information visually translates into improved decision-making, enhanced problem-solving, and ultimately, increased efficiency. This article will explore the multifaceted nature of visual matrices, delve into their applications across various domains, and provide practical strategies for leveraging their power.
This article will cover the following key areas: Understanding visual matrices, their diverse applications, analyzing the relationship between visual perception and matrix effectiveness, addressing common challenges and limitations, and finally, offering actionable tips for creating and interpreting visual matrices effectively. Readers will gain a comprehensive understanding of this powerful tool and learn how to integrate it into their workflows.
Understanding Visual Matrices: A Deep Dive
Visual matrices, also known as data matrices or simply matrices, are tables that organize data points into rows and columns. Each cell at the intersection of a row and column represents a specific data point, allowing for a structured and visually accessible representation of information. This simple structure belies the complexity of information that can be effectively displayed. The choice of what constitutes a row and column is crucial and depends heavily on the nature of the data and the insights one wishes to glean.
The power of visual matrices lies in their ability to represent multi-dimensional data effectively. Unlike simpler charts or graphs that might only depict one or two variables, matrices can display relationships between numerous variables simultaneously. This ability to visualize complex interdependencies is what makes them such a valuable tool for analysis and decision-making.
Types of Visual Matrices:
Several types of visual matrices cater to different data types and analytical needs:
- Simple Data Matrices: These matrices display numerical or categorical data directly. Each cell contains a single value.
- Heatmaps: These matrices use color gradients to represent data values, providing an immediate visual cue of high and low values. This is particularly useful for identifying trends and patterns.
- Contingency Tables: These are specifically used in statistical analysis to display the frequency distribution of two or more categorical variables. They are invaluable in exploring relationships between variables.
- Correlation Matrices: These matrices represent the correlation coefficients between different variables. They visualize the strength and direction of linear relationships between variables.
Diverse Applications of Visual Matrices
The versatility of visual matrices extends across a wide range of fields:
- Business Intelligence: Analyzing sales data, market trends, customer segmentation, and competitive landscapes.
- Market Research: Visualizing survey results, customer preferences, and market dynamics.
- Project Management: Tracking project progress, resource allocation, and task dependencies. Gantt charts are a specialized form of visual matrix.
- Scientific Research: Analyzing experimental results, gene expression data, and ecological patterns.
- Financial Analysis: Displaying investment portfolios, risk assessments, and financial performance.
Visual Perception and Matrix Effectiveness: A Synergistic Relationship
The effectiveness of a visual matrix is heavily reliant on principles of visual perception. Several factors influence how easily and accurately people interpret the information presented:
- Color Choice: Consistent and meaningful color schemes are essential, particularly in heatmaps, to avoid misleading interpretations.
- Data Labeling: Clear and concise labels for rows, columns, and data points are crucial for understanding the context of the data.
- Visual Hierarchy: Using size, color, and font variations to highlight important data points helps guide the viewer's attention.
- Matrix Size: Overly large matrices can be overwhelming, while overly small ones can lack detail. Finding the optimal size is key.
Challenges and Limitations of Visual Matrices
While visual matrices offer many benefits, some challenges must be acknowledged:
- Data Volume: Extremely large datasets can be difficult to represent effectively in a matrix format.
- Complexity: Matrices displaying many variables can become difficult to interpret, requiring careful design and labeling.
- Misinterpretation: Inaccurate or poorly designed matrices can lead to incorrect conclusions.
- Software Limitations: Not all software packages can effectively handle and visualize complex matrices.
Creating and Interpreting Visual Matrices: Actionable Strategies
To maximize the effectiveness of visual matrices, consider these strategies:
- Define Your Objective: Clearly outline the insights you aim to extract from the data.
- Choose the Right Matrix Type: Select the matrix type that best suits your data and analytical goals.
- Data Cleaning and Preparation: Ensure your data is accurate, consistent, and appropriately scaled.
- Visual Design: Employ clear labeling, consistent color schemes, and appropriate visual hierarchy.
- Iterative Refinement: Refine the matrix design based on initial interpretations and feedback.
Key Takeaways: Essential Insights into Visual Matrices
Insight | Description |
---|---|
Visual Clarity is Paramount | Clear design and labeling are critical for accurate interpretation. |
Matrix Type Selection is Crucial | The choice of matrix type directly impacts the effectiveness of the visualization. |
Data Preparation is Essential | Clean, accurate data is the foundation of a meaningful visual matrix. |
Consider Cognitive Load | Avoid overwhelming the viewer with excessive data or complexity. |
Iterative Refinement Improves Results | Continuous improvement of the matrix design enhances understanding and insight extraction. |
Exploring the Connection Between Data Storytelling and Visual Matrices
Data storytelling involves transforming raw data into compelling narratives that resonate with the audience. Visual matrices play a crucial role in this process by providing a visually engaging and easily digestible format for complex data. Effective data storytelling using matrices requires careful consideration of the narrative arc. The matrix should not only present data but also guide the viewer through a clear and logical progression of insights. This is achieved through strategic design choices like highlighting key findings, using color effectively, and providing contextual information.
Roles and Real-World Examples:
- Marketing: A heatmap visualizing customer segments based on demographics and purchase history can be a powerful tool for targeted marketing campaigns.
- Finance: A correlation matrix showing the relationships between different asset classes can inform investment strategies.
Risks and Mitigations:
- Oversimplification: Presenting complex data in an overly simplified matrix can lead to a loss of critical information.
- Misrepresentation: Poorly designed matrices can mislead the audience.
Impact and Implications:
By effectively combining visual matrices with data storytelling techniques, organizations can make more informed decisions, improve communication, and drive innovation.
Reinforcing the Connection in the Conclusion
The symbiotic relationship between visual matrices and data storytelling cannot be overstated. Visual matrices provide the structural framework, while data storytelling adds context, meaning, and narrative flow. Understanding this synergy is essential for effectively communicating complex data insights across diverse audiences.
Deeper Dive into Data Storytelling
Data storytelling, a process that transforms raw data into a compelling narrative, is crucial for effective communication. It goes beyond simply presenting data; it involves building a story around the data, establishing a context, highlighting key insights, and drawing meaningful conclusions. A strong data story should have a clear beginning, middle, and end, much like any other narrative.
Cause-and-Effect Analysis:
By strategically visualizing data through matrices, the cause-and-effect relationships within the dataset can be clearly articulated. For example, a time-series matrix depicting sales figures over time can reveal the impact of marketing campaigns or seasonal trends.
Industry Examples:
- Healthcare: Visualizing patient data through matrices can reveal patterns in disease outbreaks, treatment efficacy, and risk factors.
- Education: Matrices showing student performance data across different subjects can highlight areas needing improvement and inform pedagogical strategies.
Frequently Asked Questions (FAQ)
Q1: What software is best for creating visual matrices?
A1: Many software packages can create visual matrices, including spreadsheet programs like Microsoft Excel and Google Sheets, statistical software like R and SPSS, and data visualization tools like Tableau and Power BI. The best choice depends on your specific needs and technical skills.
Q2: How can I avoid misinterpreting a visual matrix?
A2: Carefully review the data labels, color scales, and any accompanying notes. Consider the context of the data and ensure your interpretation aligns with the overall narrative.
Q3: What are some common mistakes to avoid when creating visual matrices?
A3: Avoid using too many variables, unclear labels, inconsistent color schemes, and neglecting proper data scaling.
Q4: Can visual matrices be used for predictive modeling?
A4: While not directly used for predictive modeling themselves, matrices can display the data used to train and validate predictive models, aiding in the interpretation of model performance.
Q5: Are visual matrices suitable for all types of data?
A5: Visual matrices are most effective for structured data that can be organized into rows and columns. Unstructured data may require pre-processing before being represented in a matrix.
Q6: How can I improve my skills in interpreting visual matrices?
A6: Practice regularly, review examples of effective matrix designs, and seek feedback on your own creations. Consider taking online courses or workshops on data visualization and analysis.
Actionable Tips for Mastering Visual Matrices
- Start with a clear objective: Define the specific insights you want to glean from your data.
- Choose the appropriate matrix type: Select the type of matrix that best aligns with your data and analytical goals.
- Data cleaning is crucial: Ensure your data is accurate, complete, and consistent.
- Design for clarity: Use clear labels, a consistent color scheme, and an effective visual hierarchy.
- Iterate and refine: Don't be afraid to revise your matrix design based on initial feedback and interpretations.
- Context is key: Always provide sufficient context to help viewers understand the data presented.
- Consider your audience: Tailor the complexity and visual design of your matrix to the knowledge and understanding of your audience.
- Utilize interactive features: If possible, incorporate interactive elements to allow viewers to explore the data more deeply.
Conclusion
Visual matrices are a powerful tool for visualizing and interpreting complex data. By understanding their capabilities, limitations, and the principles of effective design and data storytelling, individuals and organizations can unlock significant value in various fields. The ability to effectively communicate complex information visually will continue to be a critical skill in our increasingly data-driven world. Embracing the power of visual matrices is not just a trend; it's a crucial step towards making data-driven decisions and driving meaningful change. The ongoing evolution of data visualization techniques will undoubtedly continue to refine and expand the applications of visual matrices, making them an even more potent tool in the future.
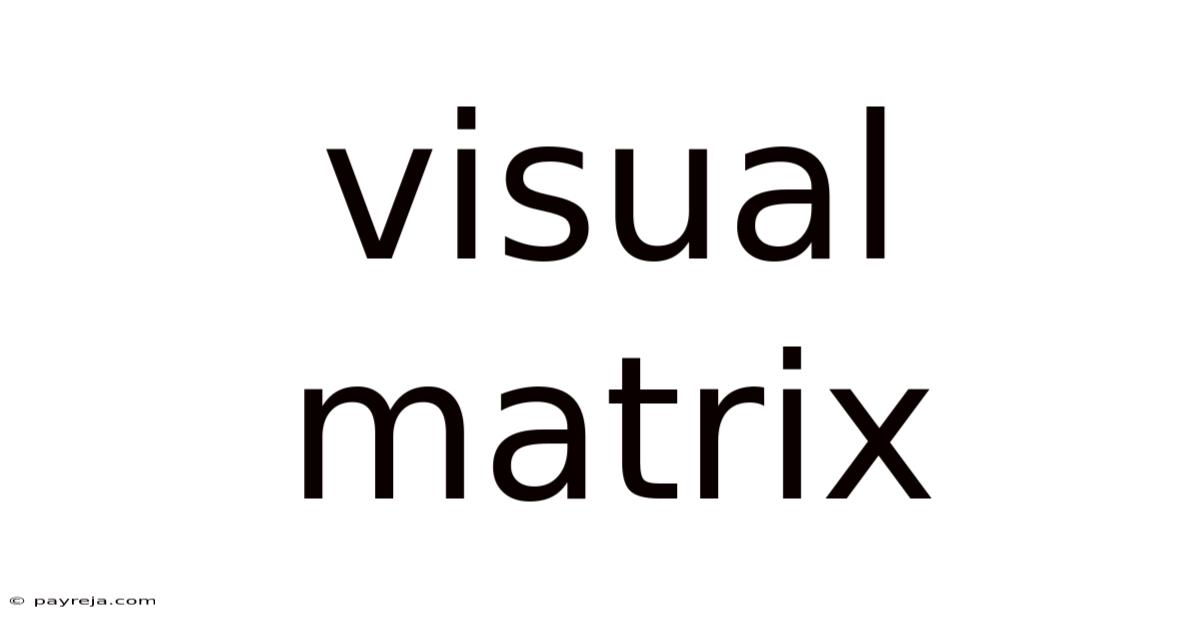
Thank you for visiting our website wich cover about Visual Matrix. We hope the information provided has been useful to you. Feel free to contact us if you have any questions or need further assistance. See you next time and dont miss to bookmark.
Also read the following articles
Article Title | Date |
---|---|
High Release Of Dopamine | Apr 23, 2025 |
E Leadership Pdf | Apr 23, 2025 |
Zoho Crm Features List | Apr 23, 2025 |
Types Of Crm Platforms | Apr 23, 2025 |
Source Engine Code | Apr 23, 2025 |