Time Series Crm Analytics
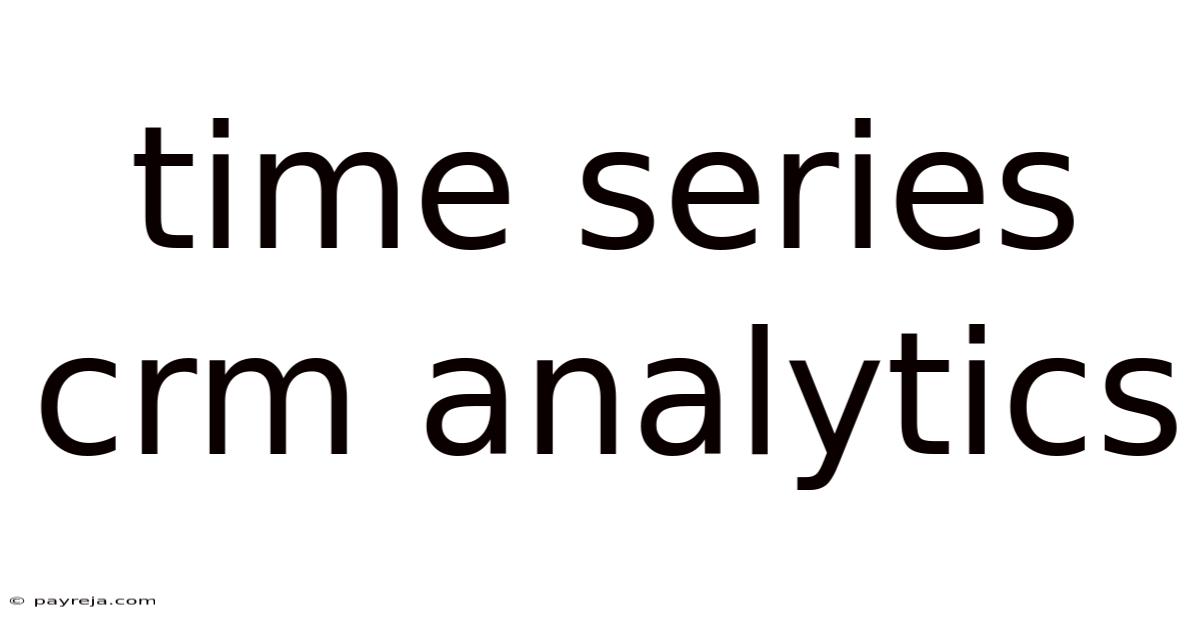
Discover more detailed and exciting information on our website. Click the link below to start your adventure: Visit Best Website meltwatermedia.ca. Don't miss out!
Table of Contents
Unlocking Growth: The Power of Time Series CRM Analytics
What if harnessing the power of time series CRM analytics could unlock unprecedented growth for your business? This transformative approach is already revolutionizing how companies understand customer behavior and optimize their strategies.
Editor’s Note: This article on Time Series CRM Analytics has been updated today to reflect the latest trends and best practices.
Time series CRM analytics represents a significant leap forward in understanding customer behavior. Instead of relying on static snapshots of data, this approach analyzes customer interactions over time, revealing patterns and trends otherwise invisible. This allows businesses to make more informed decisions, predict future behavior, and ultimately, drive revenue growth and enhance customer loyalty. The implications span various industries, from e-commerce and SaaS to finance and healthcare, impacting customer acquisition, retention, and lifetime value. Understanding the nuances of time series data within a CRM context empowers businesses to personalize experiences, optimize marketing campaigns, and proactively address potential churn.
This article will explore the core components of time series CRM analytics, including data collection, analysis techniques, practical applications, and crucial considerations. Readers will gain a comprehensive understanding of this powerful tool and learn how to leverage it for substantial business benefits.
Key Topics Covered:
- Defining Time Series CRM Analytics
- Data Sources and Preparation
- Essential Analysis Techniques
- Real-World Applications and Case Studies
- Challenges and Mitigation Strategies
- The Interplay of Time Series Analytics and Predictive Modeling
What You Will Learn:
- How to identify valuable time series data within your CRM.
- The various analytical techniques applicable to CRM time series data.
- Strategies for implementing time series analytics within your business.
- How to interpret results and translate them into actionable insights.
- The future trends and potential of time series CRM analytics.
Understanding Time Series CRM Analytics:
Time series data, by definition, is data collected and recorded over a period. In a CRM context, this includes customer interactions such as website visits, email opens, purchase history, support tickets, and social media engagements, all timestamped and tracked chronologically. Analyzing this data reveals patterns in customer behavior over time, such as seasonal purchasing habits, the impact of marketing campaigns on engagement, and the lifecycle of customer relationships. This granular level of insight allows for more precise targeting, optimized resource allocation, and a deeper understanding of customer lifetime value (CLTV).
Data Sources and Preparation:
The foundation of effective time series CRM analytics lies in the quality and completeness of your data. Data sources typically include:
- CRM System: The primary source, containing customer interactions, purchase history, and demographic data.
- Website Analytics: Data on website traffic, user behavior, and conversion rates.
- Marketing Automation Platforms: Information on email campaigns, social media interactions, and advertising performance.
- Customer Support Systems: Data on support tickets, resolution times, and customer satisfaction scores.
Data preparation is a critical step. This involves:
- Data Cleaning: Identifying and handling missing values, outliers, and inconsistencies.
- Data Transformation: Converting data into a suitable format for analysis, often involving aggregation and normalization.
- Feature Engineering: Creating new variables from existing data to enhance predictive power.
Essential Analysis Techniques:
Several analytical techniques are employed in time series CRM analytics:
- Trend Analysis: Identifying long-term patterns and trends in customer behavior.
- Seasonality Analysis: Detecting recurring patterns at regular intervals (e.g., monthly, yearly).
- Autocorrelation Analysis: Measuring the correlation between data points at different time lags.
- Moving Average: Smoothing out short-term fluctuations to reveal underlying trends.
- Exponential Smoothing: A forecasting method that assigns exponentially decreasing weights to older data points.
- ARIMA Modeling: A sophisticated statistical model used for forecasting time series data.
Real-World Applications and Case Studies:
The applications of time series CRM analytics are vast and impactful:
- Churn Prediction: Identifying customers at risk of churning by analyzing their interaction patterns over time. Early warning allows for proactive interventions, such as personalized offers or targeted support.
- Campaign Optimization: Evaluating the effectiveness of marketing campaigns by tracking customer engagement over time. This data-driven approach allows for adjustments and optimization of future campaigns.
- Sales Forecasting: Predicting future sales by analyzing historical sales data and identifying seasonal patterns or trends. This improves inventory management and resource allocation.
- Customer Segmentation: Creating more refined customer segments based on their behavior over time, enabling targeted marketing and personalized experiences.
- Product Development: Identifying product usage patterns and trends to inform future product development and innovation.
Example Case Study: An e-commerce company used time series analysis of customer purchase history to identify a significant drop in sales during specific months. By analyzing the data further, they discovered a correlation with a competitor's promotional campaigns. This insight allowed them to proactively adjust their pricing and promotions to mitigate the impact and maintain market share.
Challenges and Mitigation Strategies:
Despite its potential, time series CRM analytics faces several challenges:
- Data Quality: Incomplete or inaccurate data can lead to unreliable results. Robust data cleaning and validation processes are essential.
- Data Volume: Analyzing large datasets requires significant computational resources and expertise. Cloud-based solutions and advanced analytical tools can alleviate this challenge.
- Complexity: Implementing and interpreting time series models can be complex, requiring specialized skills and knowledge. Collaboration with data scientists or consultants may be necessary.
- Privacy Concerns: Analyzing customer data requires careful consideration of privacy regulations and ethical implications. Data anonymization and secure data handling practices are crucial.
The Interplay of Time Series Analytics and Predictive Modeling:
Time series analytics forms a crucial component of predictive modeling within CRM. By combining historical data patterns with other relevant variables (demographics, purchase history, customer support interactions), businesses can build sophisticated predictive models to forecast future behavior with greater accuracy. This allows for proactive customer engagement, personalized offers, and optimized resource allocation.
Key Insights Summary:
Insight | Description |
---|---|
Enhanced Customer Understanding | Deeper insights into customer behavior over time, revealing hidden patterns and trends. |
Proactive Churn Management | Identify at-risk customers and intervene before churn occurs. |
Optimized Marketing Campaigns | Improve campaign effectiveness by analyzing engagement patterns and optimizing future strategies. |
Accurate Sales Forecasting | Predict future sales with greater accuracy, enhancing inventory management and resource allocation. |
Personalized Customer Experiences | Create highly targeted and personalized customer experiences based on individual behavior. |
Improved Customer Lifetime Value (CLTV) | Maximize customer value by understanding their lifecycle and tailoring interactions accordingly. |
Exploring the Connection Between Predictive Modeling and Time Series CRM Analytics:
Predictive modeling relies heavily on historical data to predict future outcomes. Time series CRM analytics provides this crucial historical data, capturing the temporal dynamics of customer behavior. For instance, a model predicting customer churn might incorporate time series data on purchase frequency, website visits, and customer support interactions to create a more precise and nuanced prediction. The strength of this connection lies in its ability to account for temporal dependencies, leading to more accurate and actionable forecasts.
Roles and Real-World Examples:
- Marketing: Predictive models, leveraging time series data on email open rates and website clicks, can identify the most effective marketing channels and personalize messaging.
- Sales: Forecasting models using time series sales data can optimize inventory management and resource allocation, preventing stockouts or overstocking.
- Customer Support: Predictive models, incorporating time series data on support ticket volume and resolution times, can help optimize staffing levels and improve customer service efficiency.
Risks and Mitigations:
- Overfitting: Models might become too complex and tailored to the historical data, performing poorly on new data. Regular model validation and cross-validation techniques are crucial.
- Data Bias: Biased historical data can lead to biased predictions. Careful data cleaning and preprocessing are necessary to address this issue.
- Lack of Interpretability: Complex models might be difficult to interpret, making it challenging to understand the underlying drivers of predictions. Model explainability techniques can help address this.
Impact and Implications:
The effective use of time series CRM analytics can lead to:
- Increased Revenue: By optimizing marketing, sales, and customer service processes.
- Improved Customer Loyalty: By providing personalized and proactive customer experiences.
- Reduced Costs: By optimizing resource allocation and preventing churn.
- Enhanced Business Agility: By enabling quicker responses to market changes and customer needs.
Reinforcing the Connection in the Conclusion:
The synergistic relationship between predictive modeling and time series CRM analytics is undeniable. Time series data provides the rich, temporal context necessary for building accurate and actionable predictive models. Understanding this interconnectedness is critical for businesses seeking to leverage data-driven insights to optimize operations and drive growth. The future of CRM will undoubtedly be shaped by the continued advancement and application of these powerful analytical techniques.
Dive Deeper into Predictive Modeling:
Predictive modeling uses statistical techniques to forecast future outcomes based on historical data and other relevant variables. In the context of CRM, it can be used to predict customer churn, sales, and marketing campaign effectiveness. The models range from simple regression models to sophisticated machine learning algorithms, each with its own strengths and weaknesses. The selection of the appropriate model depends on the specific business problem, the available data, and the desired level of accuracy.
Frequently Asked Questions (FAQ):
-
Q: What are the benefits of using time series CRM analytics? A: Improved customer understanding, proactive churn management, optimized marketing campaigns, accurate sales forecasting, and personalized customer experiences.
-
Q: What type of data is needed for time series CRM analytics? A: Timestamped data on customer interactions, including website visits, email opens, purchase history, support tickets, and social media engagements.
-
Q: What are some common challenges in implementing time series CRM analytics? A: Data quality, data volume, complexity, and privacy concerns.
-
Q: What are some popular analytical techniques used in time series CRM analytics? A: Trend analysis, seasonality analysis, autocorrelation analysis, moving average, exponential smoothing, and ARIMA modeling.
-
Q: How can I ensure the accuracy of my time series CRM analytics? A: Through robust data cleaning and validation, appropriate model selection, and rigorous testing.
-
Q: What are the ethical considerations involved in using time series CRM analytics? A: Data privacy, data security, and transparency are critical ethical considerations.
Actionable Tips on Time Series CRM Analytics:
- Invest in data quality: Ensure accurate and complete data collection.
- Choose the right analytical techniques: Select methods appropriate for your specific business problem and data characteristics.
- Collaborate with data scientists: Leverage expertise to implement and interpret complex models.
- Start small and scale gradually: Begin with a pilot project focusing on a specific business challenge.
- Regularly monitor and evaluate results: Track model performance and make adjustments as needed.
- Prioritize data security and privacy: Implement robust security measures and comply with relevant regulations.
- Focus on actionable insights: Translate analytical results into practical strategies and actions.
- Embrace continuous improvement: Regularly review and refine your analytical processes to enhance accuracy and efficiency.
Strong Final Conclusion:
Time series CRM analytics offers a powerful lens through which to understand and engage with customers. By harnessing the power of historical data and advanced analytical techniques, businesses can gain invaluable insights into customer behavior, optimize their strategies, and unlock unprecedented growth opportunities. The ability to predict, personalize, and proactively address customer needs is the key to success in today's competitive landscape. Embracing time series CRM analytics is not merely an advantage; it's a necessity for sustained growth and customer-centricity. The journey toward leveraging this powerful tool starts with a commitment to data quality, a willingness to embrace new technologies, and a focus on translating data-driven insights into actionable strategies.
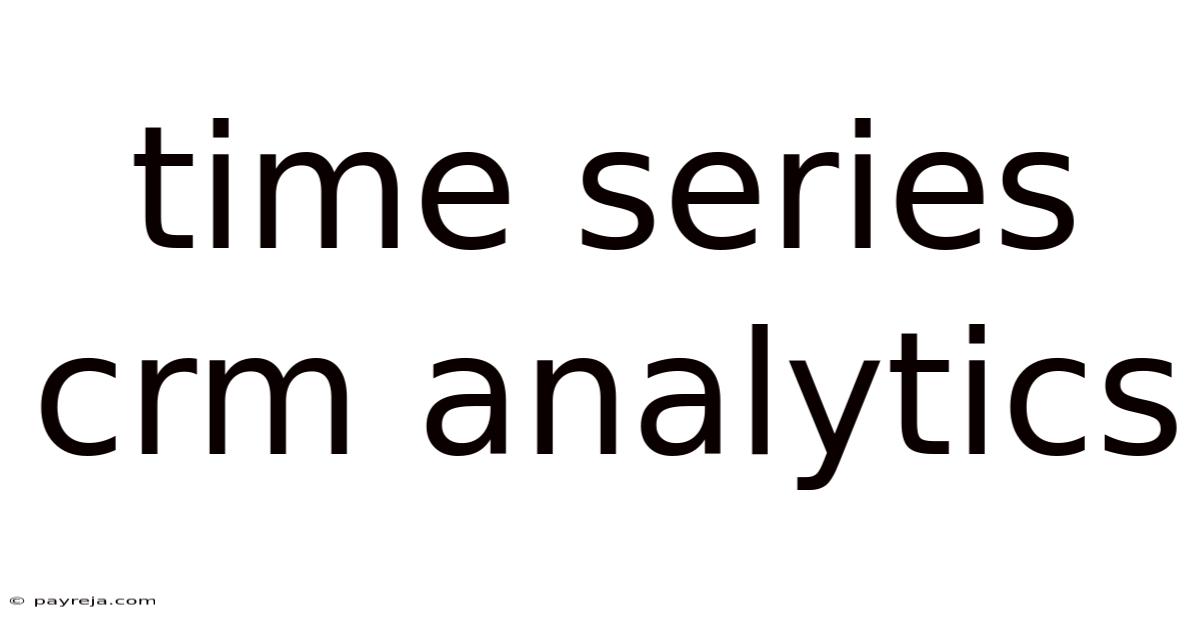
Thank you for visiting our website wich cover about Time Series Crm Analytics. We hope the information provided has been useful to you. Feel free to contact us if you have any questions or need further assistance. See you next time and dont miss to bookmark.
Also read the following articles
Article Title | Date |
---|---|
Crm Recruitment Jobs | Apr 11, 2025 |
Crimson Tide | Apr 11, 2025 |
Customer Service Modules In Crm Systems Provide Tools For | Apr 11, 2025 |
Flowchart Sales Process | Apr 11, 2025 |
App Crmincloud | Apr 11, 2025 |