Sweepiking
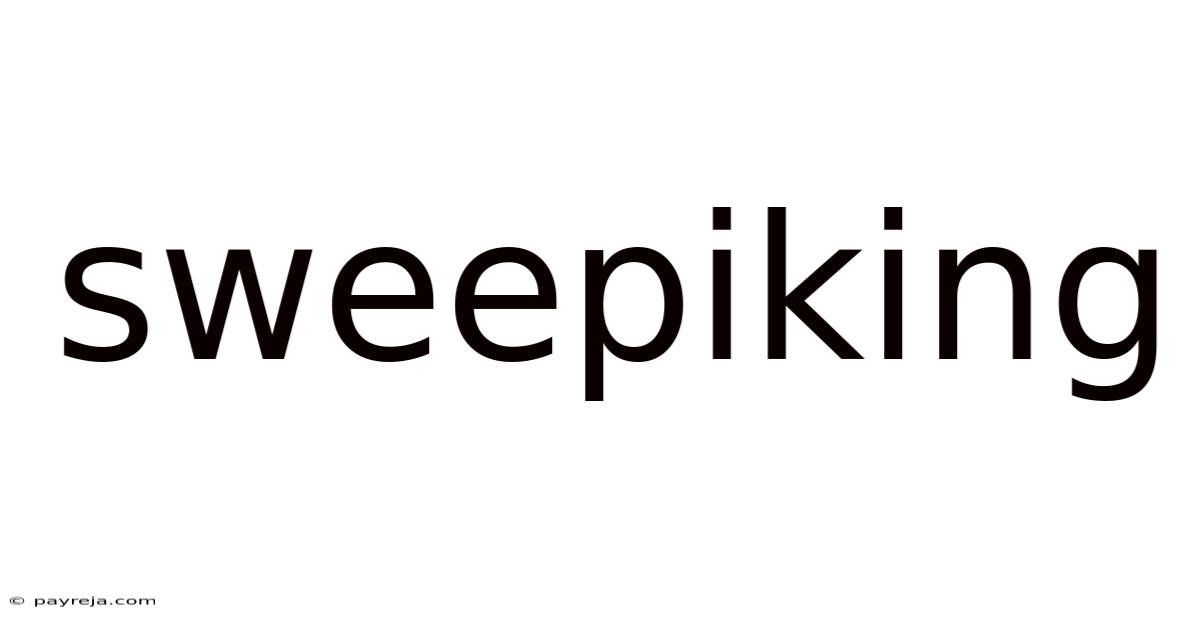
Discover more detailed and exciting information on our website. Click the link below to start your adventure: Visit Best Website meltwatermedia.ca. Don't miss out!
Table of Contents
Unlocking the Secrets of Sweepiking: A Deep Dive into This Emerging Trend
What if mastering the art of sweepiking could revolutionize your approach to data analysis and decision-making? This powerful technique is already transforming industries and unveiling hidden patterns in complex datasets.
Editor’s Note: This article on sweepiking has been published today, providing readers with the most up-to-date insights and analysis on this rapidly evolving field.
Sweepiking, a relatively new term in the field of data analysis, refers to a dynamic and iterative approach to exploring high-dimensional datasets. It combines elements of data sweeping, dimensionality reduction techniques, and intelligent pattern recognition to unveil hidden relationships and trends often missed by traditional methods. Unlike static analysis, sweepiking embraces the fluidity of data, continuously adjusting its focus and refining its insights as new information emerges. This makes it exceptionally well-suited for dealing with the ever-growing volume and complexity of big data in various industries.
This article will explore the core concepts of sweepiking, its practical applications across different sectors, the relationship between sweepiking and advanced visualization techniques, and the key considerations for successful implementation. Readers will gain a comprehensive understanding of this powerful technique and its transformative potential.
What You Will Learn:
- The fundamental principles and techniques of sweepiking.
- Real-world examples and case studies illustrating its applications.
- How sweepiking interacts with advanced visualization methods.
- The challenges and potential pitfalls of implementing sweepiking.
- Actionable strategies for leveraging sweepiking in your own data analysis projects.
Sweepiking: Unveiling Hidden Patterns in Complex Data
Sweepiking's core strength lies in its ability to handle the "curse of dimensionality," a common challenge in analyzing large datasets with numerous variables. Traditional methods often struggle with this, losing accuracy and efficiency as the number of dimensions increases. Sweepiking addresses this by employing a multi-stage process:
-
Data Sweeping: This initial phase involves systematically exploring the dataset along different dimensions. It's similar to a sweeping search, examining each variable and its interactions with others. This process often utilizes dimensionality reduction techniques like Principal Component Analysis (PCA) or t-distributed Stochastic Neighbor Embedding (t-SNE) to reduce the complexity without significant information loss.
-
Pattern Recognition: As the data is swept, sophisticated algorithms identify patterns, correlations, and anomalies. These algorithms can range from simple linear regressions to more complex machine learning models, depending on the nature of the data and the analytical goals.
-
Iterative Refinement: Unlike static analysis, sweepiking is iterative. The identified patterns guide further exploration. The analyst may choose to focus on specific sub-sections of the data, adjust parameters of the algorithms, or incorporate additional variables based on the emerging insights. This iterative process refines the analysis, revealing progressively deeper understandings.
-
Visualization and Interpretation: The final step involves visualizing the discovered patterns and interpreting their meaning within the context of the problem. Effective visualization is crucial for communicating the insights gleaned from the sweepiking process to stakeholders.
Sweepiking in Action: Real-World Applications
Sweepiking finds applications across a wide range of industries:
-
Financial Modeling: Identifying hidden correlations between various economic indicators and predicting market trends. For example, a financial institution could use sweepiking to analyze a vast dataset of economic data, market sentiment, and consumer behavior to predict stock prices with greater accuracy.
-
Medical Diagnostics: Analyzing patient data to identify subtle patterns indicative of specific diseases. Sweepiking could help detect early signs of illness by analyzing medical images, genomic data, and patient history to identify previously unseen correlations.
-
Fraud Detection: Identifying fraudulent transactions by uncovering unusual patterns in financial data. Sweepiking can be used to analyze transaction records, identifying anomalies that could indicate fraudulent activities.
-
Supply Chain Optimization: Analyzing supply chain data to improve efficiency and reduce costs. By analyzing factors like transportation costs, inventory levels, and production schedules, sweepiking can help identify bottlenecks and optimize logistics.
-
Marketing Analytics: Analyzing customer data to personalize marketing campaigns and improve conversion rates. Sweepiking can be used to identify customer segments with similar behaviors and tailor marketing strategies accordingly.
The Interplay of Sweepiking and Advanced Visualization
Effective visualization is inextricably linked to sweepiking's success. The complexity of the datasets and the iterative nature of the analysis demand powerful visualization tools. Interactive dashboards, network graphs, and heatmaps are frequently used to display the results. These visualizations allow analysts to explore the data interactively, refining their analysis and gaining deeper insights. For example, a network graph could visually represent relationships between different variables uncovered during the sweepiking process.
Key Challenges and Mitigations in Sweepiking
While sweepiking offers significant advantages, several challenges need to be addressed:
-
Computational Complexity: Analyzing large, high-dimensional datasets can be computationally intensive. Efficient algorithms and optimized hardware are necessary to manage this complexity.
-
Data Preprocessing: Data cleaning and preprocessing are crucial steps. Inaccurate or incomplete data can lead to misleading insights.
-
Interpretability: The complexity of the algorithms used in sweepiking can make it difficult to interpret the results. Explainable AI (XAI) techniques are essential to ensure transparency and trustworthiness.
-
Overfitting: The iterative nature of sweepiking increases the risk of overfitting, where the model performs well on the training data but poorly on new data. Careful validation and cross-validation techniques are necessary to mitigate this risk.
Connecting Sweepiking and Advanced Visualization Techniques
The connection between sweepiking and advanced visualization techniques is symbiotic. Sweepiking relies on visualization to effectively communicate its findings, while advanced visualization tools enable a deeper exploration of the data generated by sweepiking. For example, parallel coordinates plots can effectively visualize high-dimensional data, revealing patterns and clusters identified through the sweepiking process. Similarly, dimensionality reduction techniques like t-SNE are often used in conjunction with sweepiking to create easily interpretable visualizations of complex datasets.
Key Takeaways: Mastering the Art of Sweepiking
Insight | Explanation |
---|---|
Iterative nature crucial | Sweepiking's iterative process allows for continuous refinement and deeper insight discovery. |
Dimensionality reduction is essential | Techniques like PCA and t-SNE are vital for managing the complexity of high-dimensional datasets. |
Visualization is paramount | Effective visualization is critical for interpreting and communicating the results of sweepiking analyses. |
Computational resources are a key factor | Efficient algorithms and powerful hardware are necessary to handle the computational demands of sweepiking. |
Requires skilled data scientists | Successful implementation requires expertise in data analysis, machine learning, and visualization. |
Risk of overfitting requires mitigation | Robust validation and cross-validation techniques are essential to prevent overfitting and ensure generalizability. |
Interpretability is key for acceptance | Transparency and explainability of the results are vital for stakeholders to trust and accept the insights generated by sweepiking. |
Actionable Tips for Implementing Sweepiking
- Start with a clear objective: Define specific questions you want to answer with your analysis.
- Prepare your data carefully: Clean, preprocess, and validate your data to ensure accuracy.
- Choose appropriate algorithms: Select algorithms suited to the nature of your data and your analytical goals.
- Visualize your findings effectively: Use interactive dashboards and visualizations to explore and interpret results.
- Iterate and refine: Continuously refine your analysis based on the insights you uncover.
- Validate your findings: Ensure your results are robust and generalize beyond your specific dataset.
- Document your process: Maintain a detailed record of your analysis for transparency and reproducibility.
- Collaborate with experts: Leverage the expertise of data scientists and visualization specialists.
Frequently Asked Questions (FAQ)
Q1: What is the difference between sweepiking and traditional data analysis?
A1: Traditional data analysis often focuses on static analysis of a fixed dataset. Sweepiking, on the other hand, is a dynamic and iterative process, continuously refining its analysis as new information emerges.
Q2: What types of datasets are best suited for sweepiking?
A2: Sweepiking is particularly well-suited for large, high-dimensional datasets with complex relationships between variables.
Q3: What are the potential limitations of sweepiking?
A3: The main limitations include computational complexity, the need for significant data preprocessing, and the potential for overfitting.
Q4: What software or tools are commonly used for sweepiking?
A4: A variety of tools can be used, including programming languages like Python and R, along with specialized data analysis and visualization software.
Q5: How can I ensure the results of a sweepiking analysis are reliable?
A5: Thorough data preparation, rigorous validation, and careful interpretation of results are crucial for reliability.
Q6: Is sweepiking suitable for all types of businesses?
A6: While potentially beneficial across many industries, its suitability depends on the availability of relevant data, computational resources, and the expertise of the data science team. Smaller businesses with limited resources may find it challenging to implement effectively.
Conclusion
Sweepiking represents a significant advancement in data analysis techniques, offering the potential to uncover hidden patterns and insights in complex datasets that traditional methods often miss. By combining data sweeping, dimensionality reduction, and iterative refinement, sweepiking enables a more dynamic and insightful approach to understanding data. However, successful implementation requires careful consideration of computational resources, data quality, and the importance of robust validation techniques. As data continues to grow in volume and complexity, the value and importance of sweepiking will only continue to increase. The ability to harness its power will provide businesses and researchers with a significant competitive advantage in an increasingly data-driven world.
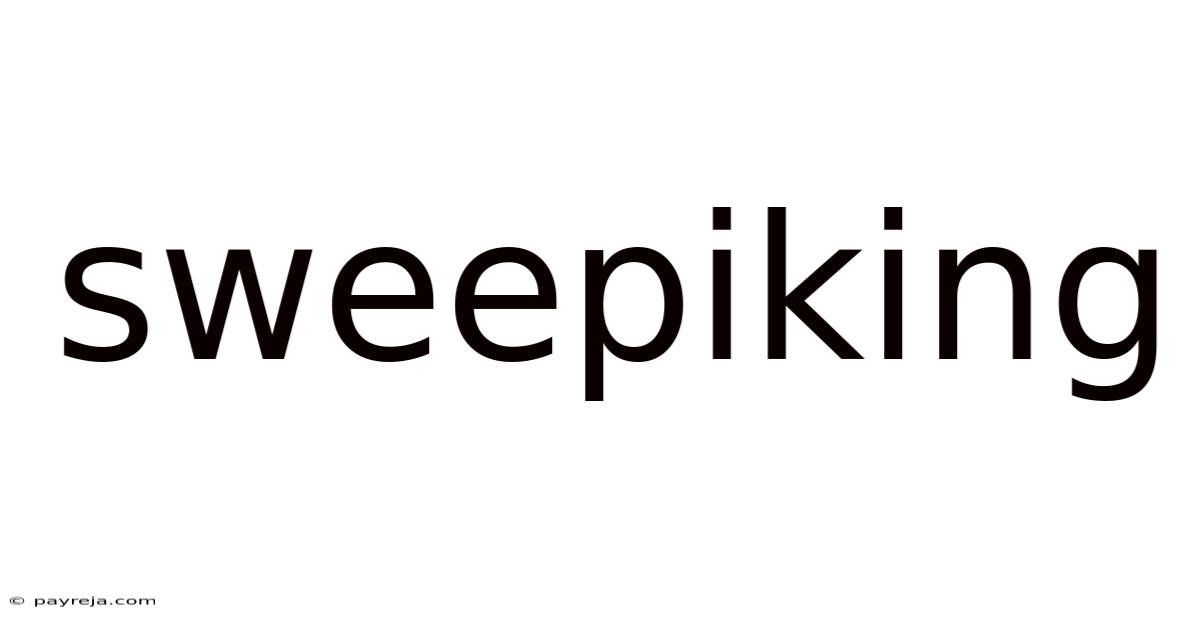
Thank you for visiting our website wich cover about Sweepiking. We hope the information provided has been useful to you. Feel free to contact us if you have any questions or need further assistance. See you next time and dont miss to bookmark.
Also read the following articles
Article Title | Date |
---|---|
What Is Braze Company | Apr 20, 2025 |
Crm 33423 | Apr 20, 2025 |
How Does Crm Help Sales | Apr 20, 2025 |
What Is The Best Crm For Real Estate Investors | Apr 20, 2025 |
S Letter Names For Boy In Telugu | Apr 20, 2025 |