Leading Mode
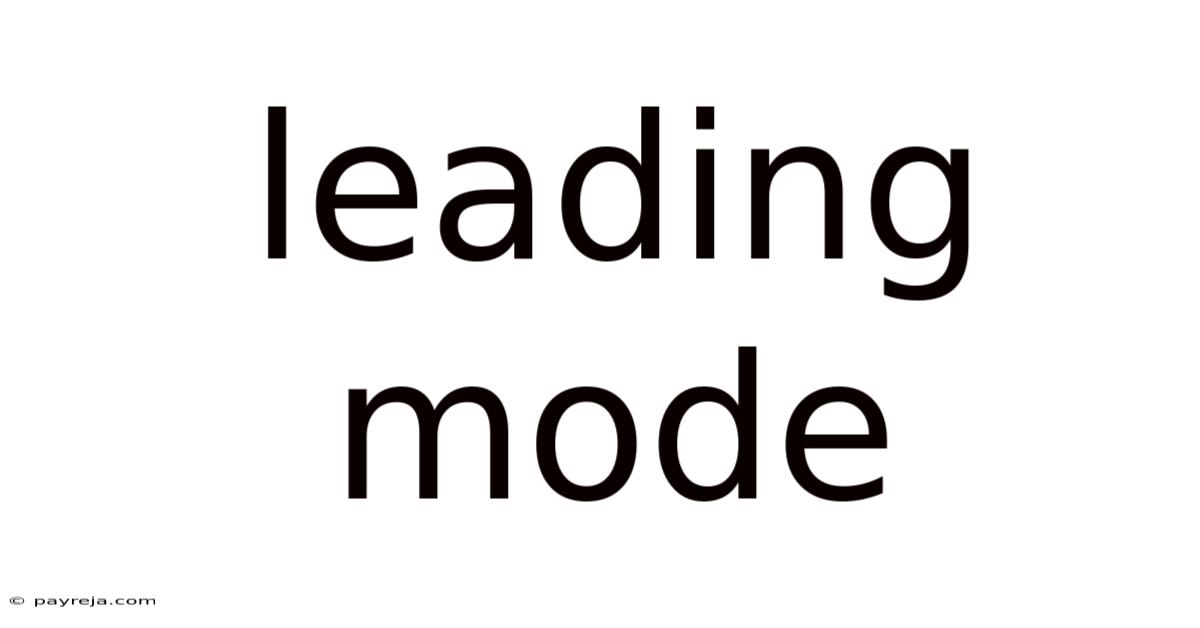
Discover more detailed and exciting information on our website. Click the link below to start your adventure: Visit Best Website meltwatermedia.ca. Don't miss out!
Table of Contents
Unlocking the Power of Leading Mode: A Deep Dive into Predictive Analytics
What if understanding leading mode could revolutionize forecasting and decision-making?
Leading mode, a powerful predictive analytics tool, is reshaping industries and offering unprecedented insights.
Editor’s Note: This article on leading mode has been published today, providing you with the most up-to-date information and insights on this transformative analytical technique.
Leading mode, often overlooked in favor of more familiar statistical measures like mean, median, and standard deviation, offers a unique and powerful approach to predictive analytics. Unlike these traditional measures that describe the central tendency of a dataset, leading mode identifies the most frequent value expected in the future, based on observed trends and patterns. This forward-looking perspective makes it invaluable across diverse fields, from finance and marketing to supply chain management and healthcare. This article explores the significance of leading mode, its practical applications, and its potential impact on various industries.
What This Article Covers:
This article will cover the definition and calculation of leading mode, its practical applications in various industries, challenges in its implementation, and the future implications of this powerful analytical technique. Readers will gain a comprehensive understanding of leading mode, enabling them to leverage its predictive power for improved decision-making. The article will also explore the relationship between leading mode and other predictive modeling techniques, providing a holistic view of its place within the broader landscape of data analysis.
Leading Mode: Definition and Calculation
Leading mode differs fundamentally from traditional mode, which identifies the most frequent value in an existing dataset. Leading mode, however, projects the most likely future most frequent value. Its calculation relies on identifying trends within time-series data. One common approach involves:
-
Data Preparation: Clean and prepare the time-series data, ensuring accuracy and consistency. This might involve handling missing values, outliers, and seasonal adjustments.
-
Trend Identification: Analyze the data to identify underlying trends using techniques like moving averages, exponential smoothing, or ARIMA models. The goal is to discern the direction and magnitude of the trend.
-
Mode Projection: Based on the identified trend, project the most likely future value that will become the most frequent. This projection considers the momentum of the trend and potential fluctuations. Sophisticated algorithms and machine learning techniques can be employed for more accurate projections, especially with complex datasets.
-
Validation and Refinement: Continuously validate the leading mode predictions against actual data and refine the model as needed. This iterative process enhances the accuracy and reliability of the predictions.
Real-World Applications of Leading Mode
The applications of leading mode are vast and impactful:
-
Finance: Predicting market trends, identifying potential investment opportunities, and managing risk more effectively. For example, analyzing historical transaction data to predict the most likely future price point for a specific asset.
-
Marketing: Forecasting customer behavior, optimizing marketing campaigns, and personalizing customer experiences. This could involve projecting the most popular product or service in a given timeframe.
-
Supply Chain Management: Optimizing inventory levels, predicting demand fluctuations, and improving supply chain efficiency. Leading mode can be used to forecast the most frequently ordered item, allowing for better stock management.
-
Healthcare: Predicting disease outbreaks, optimizing resource allocation, and improving patient outcomes. Analyzing patient data to predict the most common diagnosis or treatment plan in the future could significantly improve healthcare planning.
Challenges in Implementing Leading Mode
Despite its potential, implementing leading mode presents certain challenges:
-
Data Quality: Accurate and reliable data is crucial. Inaccurate or incomplete data can lead to flawed predictions.
-
Trend Complexity: Identifying and interpreting complex trends can be challenging, especially in volatile markets or systems.
-
Model Selection: Choosing the appropriate model for trend identification and projection depends on the specific dataset and application.
-
Computational Resources: Sophisticated algorithms for leading mode prediction often require significant computational power.
Leading Mode and Other Predictive Modeling Techniques
Leading mode is not a standalone technique. It often complements other predictive modeling methods, such as:
-
Regression Analysis: Leading mode can provide insights into the most frequent outcome, while regression analysis can model the relationships between variables influencing that outcome.
-
Time Series Analysis: Leading mode builds upon time series analysis by focusing on the future mode instead of overall trends.
-
Machine Learning: Machine learning algorithms can enhance the accuracy of leading mode prediction by learning from complex patterns in the data.
Key Insights on Leading Mode
Insight | Description |
---|---|
Forward-looking prediction | Leading mode focuses on predicting the future most frequent value, unlike traditional mode. |
Trend identification is crucial | Accurate trend identification is essential for reliable leading mode predictions. |
Complements other predictive methods | Leading mode works effectively in conjunction with other predictive modeling techniques for enhanced accuracy. |
Data quality is paramount | Reliable predictions depend on high-quality, accurate, and complete data. |
Continuous validation is necessary | Regularly validate and refine the leading mode model to ensure accuracy and adapt to changing trends. |
The Interplay Between Seasonality and Leading Mode
Seasonality significantly influences leading mode predictions. Understanding and accounting for seasonal patterns is crucial for accurate forecasting. For instance, if predicting ice cream sales, the leading mode prediction must factor in the typical increase in demand during summer months. Ignoring seasonality can lead to drastically inaccurate predictions.
Roles and Real-World Examples
Leading mode plays a crucial role in various industries:
-
Retail: Predicting the most popular items during specific sales periods, allowing retailers to optimize inventory and marketing efforts.
-
Manufacturing: Forecasting demand for specific components to streamline production and prevent shortages.
-
Finance: Predicting the most frequent trade volumes or price points in a specific market sector to inform investment strategies.
Risks and Mitigations
The primary risk associated with leading mode is inaccurate predictions due to poor data quality or inappropriate model selection. Mitigating these risks requires:
- Rigorous data validation and cleaning.
- Careful selection of the appropriate model based on the dataset and context.
- Continuous monitoring and refinement of the predictive model.
Impact and Implications
The accurate application of leading mode holds significant implications for various fields. It allows for proactive decision-making, improved resource allocation, and more effective risk management. It drives efficiency, optimizes processes, and ultimately contributes to improved profitability and overall success.
Reinforcing the Connection between Seasonality and Leading Mode
The relationship between seasonality and leading mode is undeniable. Seasonality introduces cyclical patterns that must be considered when projecting the future most frequent value. Ignoring these patterns will inevitably lead to inaccurate forecasts.
Diving Deeper into Seasonality
Seasonality refers to predictable, recurring fluctuations in data that occur within a fixed time period. These patterns can be daily, weekly, monthly, or yearly. Several techniques help identify and model seasonality, including:
- Decomposition methods: Separating the seasonal component from the trend and residual components in the time series data.
- Fourier analysis: Representing the seasonal component using trigonometric functions.
- Seasonal ARIMA models: Incorporating seasonality directly into the ARIMA model for forecasting.
Frequently Asked Questions (FAQ)
-
Q: What is the difference between leading mode and traditional mode?
- A: Traditional mode identifies the most frequent value in an existing dataset. Leading mode predicts the most likely future most frequent value based on identified trends.
-
Q: How accurate are leading mode predictions?
- A: The accuracy of leading mode predictions depends on several factors, including data quality, model selection, and the complexity of underlying trends. Continuous validation and model refinement are crucial for improving accuracy.
-
Q: Can leading mode be used for short-term and long-term forecasting?
- A: Yes, leading mode can be applied to both short-term and long-term forecasting, although the approach and model complexity may differ.
-
Q: What are some limitations of leading mode?
- A: Limitations include the need for high-quality data, the challenge of identifying complex trends, and the potential for computational intensity.
-
Q: How does leading mode relate to other forecasting methods?
- A: Leading mode often complements other forecasting methods, such as regression analysis and time series analysis, providing a more comprehensive and accurate predictive model.
-
Q: What software or tools are available for implementing leading mode?
- A: Various statistical software packages and programming languages (like R and Python) offer the necessary tools for data analysis, trend identification, and predictive modeling required for leading mode implementation.
Actionable Tips on Utilizing Leading Mode
- Ensure data quality: Thoroughly clean and validate your data before applying leading mode.
- Identify underlying trends: Use appropriate techniques to effectively identify and understand trends in your data.
- Select the right model: Choose a predictive model that best suits your data and forecasting needs.
- Validate and refine the model: Continuously validate and refine your model to improve accuracy and adapt to changing conditions.
- Integrate with other methods: Combine leading mode with other forecasting techniques for a more comprehensive approach.
- Visualize your results: Use charts and graphs to visualize the leading mode predictions and understand their implications.
- Account for seasonality: Consider seasonal patterns when making predictions to avoid inaccurate results.
Strong Final Conclusion
Leading mode represents a significant advancement in predictive analytics, offering a powerful tool for anticipating future trends and making data-driven decisions. By understanding its principles and applying its techniques correctly, organizations across various industries can unlock valuable insights, enhance efficiency, and gain a competitive advantage in today's dynamic marketplace. The continuous development and refinement of leading mode algorithms promise even greater predictive accuracy and wider applicability in the future. The insights gained from this article empower readers to leverage this powerful tool and reshape their approach to forecasting and strategic decision-making.
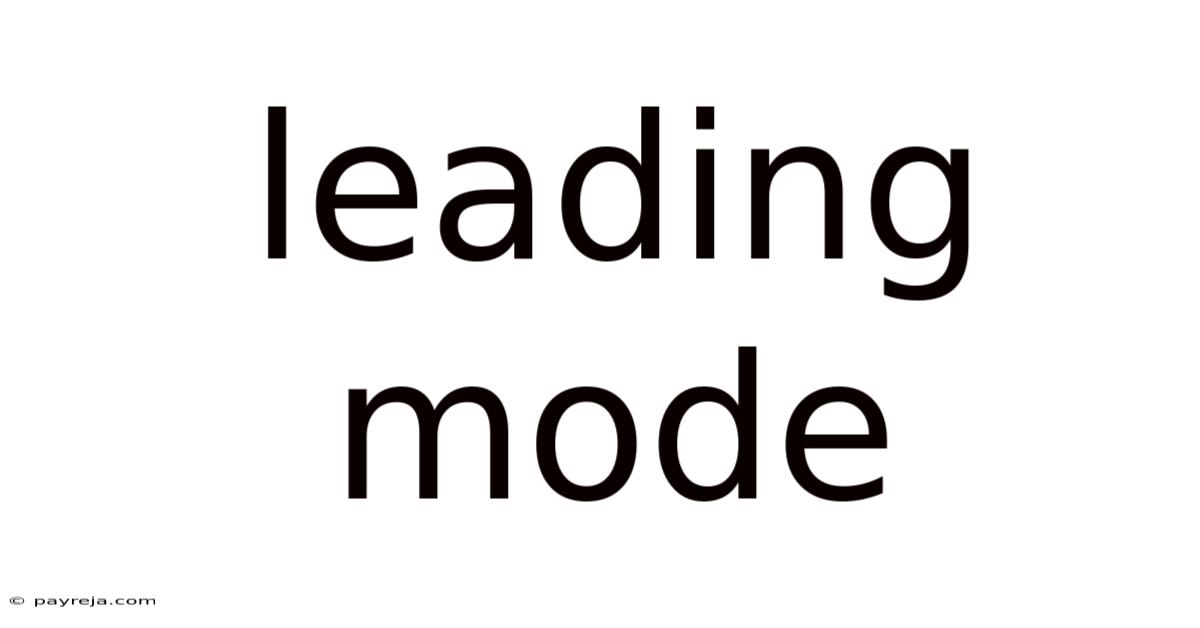
Thank you for visiting our website wich cover about Leading Mode. We hope the information provided has been useful to you. Feel free to contact us if you have any questions or need further assistance. See you next time and dont miss to bookmark.
Also read the following articles
Article Title | Date |
---|---|
Telecom Crm My Salesforce | Mar 16, 2025 |
Crm Plumbing And Heating | Mar 16, 2025 |
Salesforce Quote Process | Mar 16, 2025 |
Is Microsoft Dynamics A Crm | Mar 16, 2025 |
Peoplesoft Crm System | Mar 16, 2025 |