Digital Scoring System
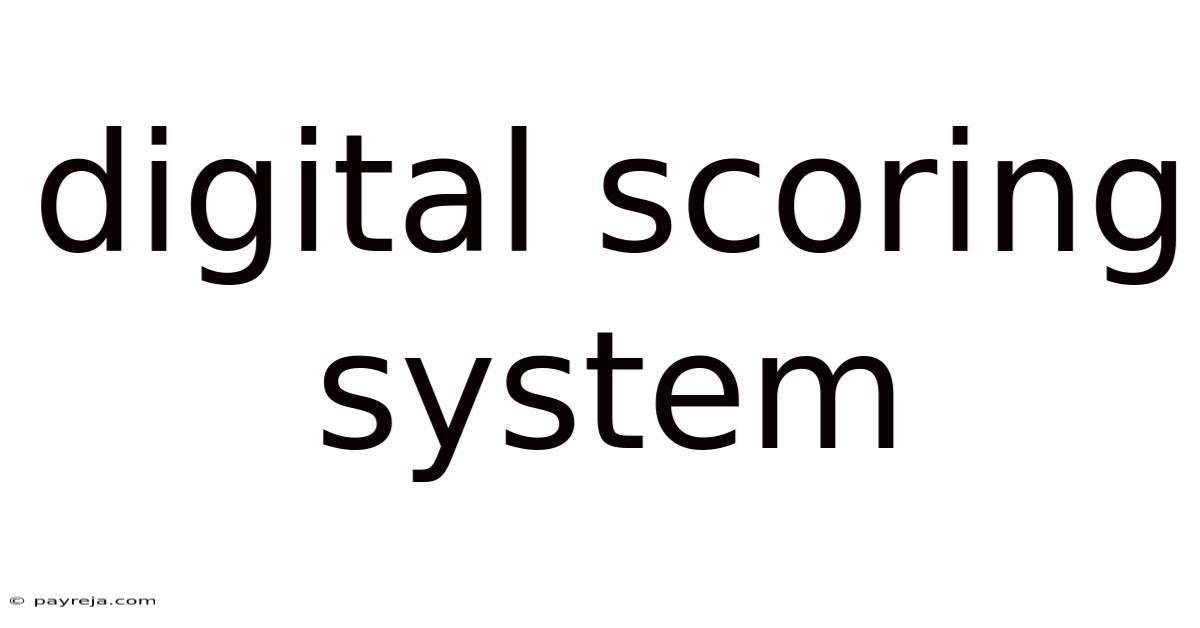
Discover more detailed and exciting information on our website. Click the link below to start your adventure: Visit Best Website meltwatermedia.ca. Don't miss out!
Table of Contents
Unveiling the Power of Digital Scoring Systems: A Comprehensive Guide
What if a perfectly objective and efficient evaluation system could revolutionize various sectors? Digital scoring systems are already transforming how we assess performance, risk, and creditworthiness across industries.
Editor’s Note: This article on digital scoring systems was published today, offering the most current insights and analysis available.
Digital scoring systems are rapidly changing how we evaluate a vast array of things, from credit risk to student performance and even employee suitability. These systems leverage sophisticated algorithms and vast datasets to generate numerical scores representing different aspects of a subject, offering a more efficient and potentially unbiased approach compared to traditional methods. This transformation has significant implications across numerous industries, impacting business operations, resource allocation, and overall decision-making.
This article will delve into the core aspects of digital scoring systems, examining their mechanics, applications, advantages, and limitations. Readers will gain a comprehensive understanding of this transformative technology, including its impact on various sectors, the ethical considerations involved, and the future trends shaping its evolution. We will explore the relationship between data quality and scoring accuracy, and provide actionable tips for effectively implementing and interpreting digital scoring results.
Understanding the Mechanics of Digital Scoring Systems
Digital scoring systems rely on complex algorithms to analyze massive datasets and generate numerical scores. These algorithms typically incorporate statistical modeling techniques, machine learning, and artificial intelligence to assess various factors contributing to a specific outcome. The process generally involves:
- Data Collection: Gathering relevant data points from diverse sources. This could include financial records, academic transcripts, behavioral data, or social media activity, depending on the application.
- Data Cleaning and Preprocessing: Cleaning the data to handle missing values, inconsistencies, and outliers. This step is crucial for accurate and reliable results.
- Feature Engineering: Selecting and transforming relevant variables into features that the algorithm can effectively utilize. This often involves sophisticated techniques to create predictive variables.
- Model Training: Training a predictive model using a portion of the data to learn the relationships between input features and the target variable (the score). Common machine learning algorithms include logistic regression, support vector machines, and neural networks.
- Model Evaluation: Assessing the performance of the trained model using metrics such as accuracy, precision, recall, and F1-score. This ensures the system's reliability and predictive power.
- Score Generation: Applying the trained model to new data to generate numerical scores for individual subjects.
- Model Monitoring and Maintenance: Regularly monitoring the model's performance and retraining it periodically to account for changes in data patterns and maintain accuracy.
Applications Across Industries
Digital scoring systems have found widespread application in numerous sectors, including:
- Finance: Credit scoring models are perhaps the most well-known example, used by lenders to assess the creditworthiness of individuals and businesses. These systems analyze factors like income, debt, and credit history to predict the likelihood of loan defaults.
- Education: Educational institutions utilize digital scoring systems to evaluate student performance, predict academic success, and personalize learning experiences. These systems can analyze test scores, assignment grades, and attendance data to provide insights into student progress.
- Human Resources: Companies employ digital scoring systems in recruitment processes to screen candidates, predict job performance, and assess cultural fit. These systems can analyze resumes, cover letters, and even social media profiles to identify suitable candidates.
- Healthcare: In healthcare, digital scoring systems are used to assess patient risk, predict disease progression, and personalize treatment plans. These systems can analyze medical records, genetic information, and lifestyle factors to provide individualized risk assessments.
- Insurance: Insurers use digital scoring systems to assess risk and determine insurance premiums. These systems consider factors like driving records, health history, and location to predict the likelihood of claims.
- Marketing: Digital scoring systems are used in marketing to segment customers, personalize advertising campaigns, and predict customer behavior. These systems can analyze purchasing history, browsing behavior, and demographics to tailor marketing messages.
Advantages and Limitations of Digital Scoring Systems
While digital scoring systems offer several advantages, it's crucial to acknowledge their limitations:
Advantages:
- Efficiency and Automation: Automate the scoring process, significantly reducing manual effort and time.
- Objectivity and Consistency: Reduce human bias in decision-making, leading to fairer and more consistent evaluations.
- Enhanced Accuracy: Leverage large datasets and sophisticated algorithms to produce more accurate predictions.
- Improved Decision-Making: Provide data-driven insights that inform better decisions across various domains.
- Scalability and Flexibility: Easily adapt to different contexts and scale to handle large volumes of data.
Limitations:
- Data Bias: If the training data reflects existing biases, the resulting scores may perpetuate and amplify those biases.
- Lack of Transparency: Complex algorithms can make it difficult to understand how scores are generated, leading to concerns about fairness and accountability.
- Overreliance on Data: May overlook important qualitative factors that cannot be easily quantified.
- Privacy Concerns: Collecting and using personal data raises significant privacy concerns.
- System Errors: Errors in data collection, processing, or algorithm design can lead to inaccurate or unfair scores.
The Interplay Between Data Quality and Scoring Accuracy
The accuracy and fairness of a digital scoring system are heavily dependent on the quality of the data used to train and operate the system. Poor data quality can lead to biased, inaccurate, and unreliable scores. Therefore, ensuring high-quality data is paramount. This involves:
- Data Validation: Verifying the accuracy and completeness of data.
- Data Cleaning: Removing errors, inconsistencies, and outliers.
- Data Transformation: Converting data into a suitable format for the algorithm.
- Data Enrichment: Supplementing existing data with additional relevant information.
Careful attention to data quality throughout the entire process is crucial for building robust and trustworthy digital scoring systems.
Key Takeaways: Understanding Digital Scoring Systems
Insight | Description |
---|---|
Efficiency and Automation | Digital scoring systems significantly automate the evaluation process, saving time and resources. |
Improved Accuracy and Objectivity | These systems offer more accurate and objective evaluations compared to traditional methods, reducing human bias. |
Broad Applicability | They find applications across diverse sectors, from finance and education to healthcare and marketing. |
Data Quality is Critical | The accuracy and fairness of the system heavily rely on the quality of the data used; poor data leads to unreliable scores. |
Ethical Considerations are Paramount | Issues of bias, transparency, privacy, and accountability need careful consideration when designing and implementing these systems. |
Continuous Monitoring is Necessary | Regular monitoring and maintenance of the model are essential to ensure accuracy and adapt to changing data patterns and evolving needs. |
The Relationship Between Data Privacy and Digital Scoring Systems
Data privacy is a major concern when implementing digital scoring systems, especially when dealing with sensitive personal information. The collection and use of personal data must comply with relevant data privacy regulations such as GDPR (General Data Protection Regulation) and CCPA (California Consumer Privacy Act). This necessitates:
- Data Minimization: Collecting only the necessary data.
- Data Security: Implementing robust security measures to protect data from unauthorized access.
- Transparency and Consent: Being transparent about how data is collected and used, obtaining explicit consent from individuals.
- Data Anonymization and Pseudonymization: Techniques to protect individual identities while still allowing for data analysis.
Striking a balance between leveraging data for accurate scoring and protecting individual privacy is essential for ethical and responsible implementation.
Exploring the Connection Between Algorithmic Bias and Digital Scoring Systems
Algorithmic bias is a significant challenge in digital scoring systems. If the training data reflects existing societal biases, the resulting algorithm may perpetuate and amplify these biases, leading to unfair or discriminatory outcomes. Mitigating algorithmic bias requires:
- Careful Data Selection: Ensuring the training data is representative of the entire population and free from biases.
- Bias Detection and Mitigation Techniques: Employing algorithms and techniques to identify and reduce bias in the data and model.
- Regular Audits and Monitoring: Regularly auditing the system for bias and making adjustments as needed.
- Explainable AI (XAI): Using techniques to make the decision-making process of the algorithm more transparent and understandable.
Dive Deeper into Algorithmic Bias
Algorithmic bias occurs when a computer system produces results that are systematically prejudiced against certain groups of people. This bias can stem from several sources:
- Biased Data: The data used to train the algorithm may already reflect societal biases. For example, if a credit scoring algorithm is trained primarily on data from a specific demographic, it may unfairly disadvantage other groups.
- Biased Features: The features selected for the algorithm may disproportionately affect certain groups. For example, using zip codes as a predictor in a loan application could disadvantage applicants from lower-income neighborhoods.
- Algorithmic Design: The algorithm itself may contain inherent biases that amplify existing biases in the data.
Addressing algorithmic bias requires a multifaceted approach involving careful data selection, bias detection techniques, and ongoing monitoring and evaluation.
Frequently Asked Questions about Digital Scoring Systems
Q1: Are digital scoring systems fair and unbiased?
A1: While digital scoring systems aim for objectivity, they can still reflect biases present in the data they are trained on. Careful attention to data quality, bias mitigation techniques, and ongoing monitoring are crucial for ensuring fairness.
Q2: How can I ensure the accuracy of a digital scoring system?
A2: Accuracy depends on data quality, algorithm selection, and rigorous testing. Regularly validating the data, employing robust algorithms, and monitoring system performance are crucial for accuracy.
Q3: What are the privacy implications of digital scoring systems?
A3: These systems often handle sensitive personal data, raising privacy concerns. Compliance with data privacy regulations, data minimization, and robust security measures are essential to mitigate these risks.
Q4: Can digital scoring systems be manipulated?
A4: Like any system, they are susceptible to manipulation if not properly secured and monitored. Robust security measures and regular audits are essential to prevent manipulation.
Q5: What is the future of digital scoring systems?
A5: Expect increased sophistication, greater transparency, and stronger focus on ethical considerations. The use of explainable AI and robust bias mitigation techniques will be crucial.
Q6: How can I interpret a digital score?
A6: The interpretation depends on the specific system and its scoring scale. The system's documentation should provide clear explanations of the score's meaning and implications.
Actionable Tips for Implementing and Interpreting Digital Scoring Systems
- Ensure Data Quality: Prioritize data cleaning, validation, and enrichment to minimize bias and errors.
- Choose Appropriate Algorithms: Select algorithms suitable for the specific application and data characteristics.
- Mitigate Algorithmic Bias: Employ bias detection and mitigation techniques throughout the development process.
- Prioritize Transparency: Document the system’s methodology and make it understandable to stakeholders.
- Monitor Performance Regularly: Continuously monitor the system’s performance and retrain the model as needed.
- Comply with Data Privacy Regulations: Adhere to all relevant data privacy laws and regulations.
- Focus on Explainability: Employ techniques to make the system's decisions more transparent and understandable.
- Seek Expert Advice: Consult with data scientists and ethicists to ensure ethical and responsible implementation.
Conclusion
Digital scoring systems are transforming decision-making across numerous sectors. While offering significant advantages in efficiency, objectivity, and accuracy, careful consideration of ethical implications, including bias, transparency, and privacy, is crucial. By addressing these challenges and focusing on responsible implementation, these powerful tools can unlock significant opportunities for innovation and progress while ensuring fairness and accountability. The future of digital scoring systems lies in their continued evolution towards greater transparency, explainability, and ethical considerations, ultimately leading to more equitable and effective evaluation processes across various industries.
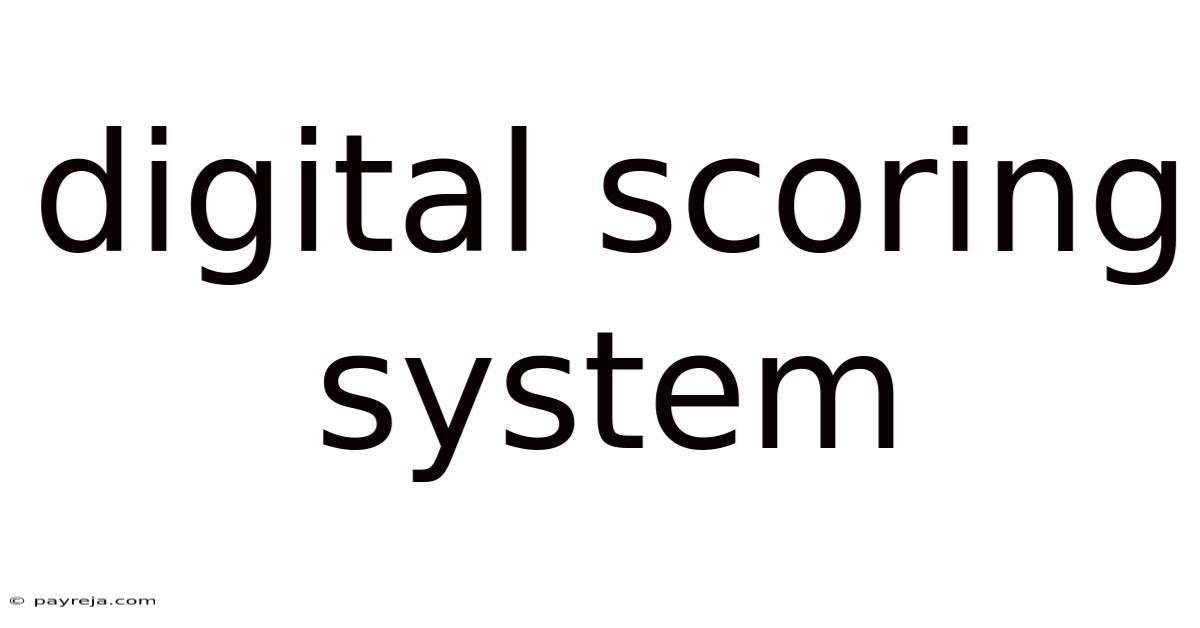
Thank you for visiting our website wich cover about Digital Scoring System. We hope the information provided has been useful to you. Feel free to contact us if you have any questions or need further assistance. See you next time and dont miss to bookmark.
Also read the following articles
Article Title | Date |
---|---|
Physician Crm | Apr 23, 2025 |
Source Connect Tech Support | Apr 23, 2025 |
Welcome Home Location | Apr 23, 2025 |
Zoho Crm For Everyone Release Date | Apr 23, 2025 |
Salesforce Database Error | Apr 23, 2025 |