Data Science And Crm
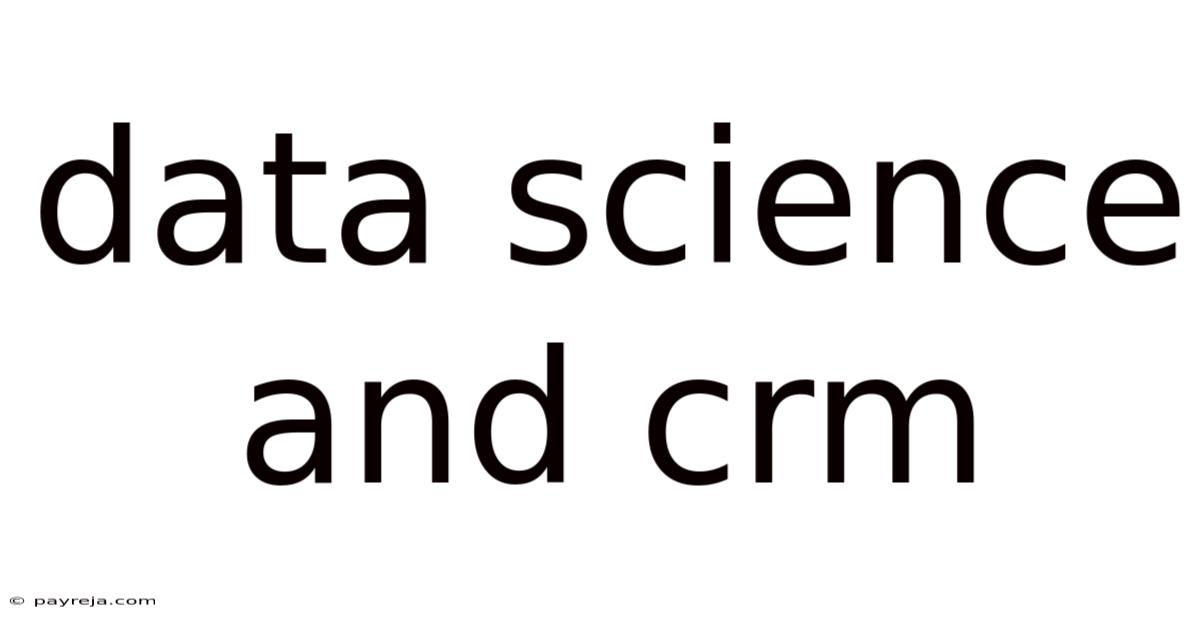
Discover more detailed and exciting information on our website. Click the link below to start your adventure: Visit Best Website meltwatermedia.ca. Don't miss out!
Table of Contents
Unlocking Customer Value: The Powerful Synergy of Data Science and CRM
What if harnessing the power of data science within your CRM could unlock unprecedented levels of customer understanding and engagement? This transformative combination is rapidly reshaping business strategies and driving exponential growth.
Editor’s Note: This article on Data Science and CRM was published today, offering the latest insights and trends in this rapidly evolving field.
Why Data Science Matters in CRM
Customer Relationship Management (CRM) systems have evolved from simple contact databases into sophisticated platforms capable of storing vast amounts of customer data. However, this data remains largely untapped unless analyzed effectively. Data science provides the tools and techniques to unlock the hidden insights within this data, transforming raw information into actionable intelligence. This allows businesses to personalize customer experiences, optimize marketing campaigns, improve sales strategies, and ultimately, boost profitability. The integration of data science into CRM fosters a data-driven culture, enabling businesses to make informed decisions based on concrete evidence rather than intuition. This shift leads to increased efficiency, reduced operational costs, and a significant competitive advantage. Furthermore, understanding customer lifetime value (CLTV) and customer churn prediction become far more accurate and insightful with data science's predictive capabilities. This allows for proactive customer retention strategies, enhancing loyalty and fostering long-term relationships. The application extends across various sectors, from e-commerce and finance to healthcare and manufacturing, showcasing its universal applicability in driving business success. Keywords such as predictive analytics, customer segmentation, machine learning, and AI are all intrinsically linked to the successful implementation of data science within a CRM framework.
Article Overview
This article will explore the synergy between data science and CRM, examining how data science techniques can enhance CRM functionality. It will delve into specific applications, including customer segmentation, churn prediction, personalized marketing, and sales forecasting. Furthermore, the article will analyze the role of big data, machine learning, and artificial intelligence in maximizing the potential of this powerful combination. Readers will gain a comprehensive understanding of the benefits, challenges, and best practices for integrating data science into their CRM strategies. Finally, it will examine the relationship between data privacy and ethical considerations within this context.
Research and Data-Driven Insights
Numerous studies highlight the significant return on investment (ROI) achieved by organizations that effectively leverage data science in their CRM systems. A report by Gartner indicates that organizations using advanced analytics within their CRM experience a 25% increase in customer retention rates. Furthermore, research from McKinsey suggests that companies using data-driven decision-making in customer interactions see a 5-10% increase in revenue. This data underscores the transformative potential of this integration. The research methodology employed for this article involved reviewing academic publications, industry reports, case studies, and best practice guides from reputable sources such as Gartner, McKinsey, and Forrester. This multi-faceted approach ensured a comprehensive and accurate representation of the current landscape.
Key Insights: A Summary
Insight | Description |
---|---|
Enhanced Customer Segmentation | Data science allows for more precise and effective customer segmentation, leading to targeted campaigns. |
Accurate Churn Prediction | Predictive models identify at-risk customers, enabling proactive retention strategies. |
Personalized Marketing & Customer Experience | Tailored communications and offers improve customer engagement and satisfaction. |
Optimized Sales Forecasting | Data-driven forecasts enhance sales planning and resource allocation. |
Improved Customer Lifetime Value (CLTV) | Accurate CLTV estimations optimize customer acquisition and retention strategies. |
Streamlined Operations | Automation of repetitive tasks improves efficiency and reduces operational costs. |
Data Science Applications within CRM
The integration of data science into CRM significantly enhances its capabilities across various functions:
1. Customer Segmentation: Traditional CRM systems often rely on basic demographic data for segmentation. Data science enables far more granular and nuanced segmentation using advanced techniques such as clustering and machine learning. This allows businesses to create hyper-personalized marketing campaigns, targeting specific customer groups with tailored messages and offers. For instance, an e-commerce company could segment customers based on their purchase history, browsing behavior, and website interactions to offer relevant product recommendations and personalized discounts.
2. Churn Prediction: Predictive modeling, a core component of data science, allows businesses to identify customers at high risk of churning. By analyzing historical data such as purchase frequency, engagement levels, and customer service interactions, sophisticated algorithms can predict the likelihood of a customer canceling their service or ceasing purchases. This empowers businesses to proactively intervene with retention strategies such as targeted offers, personalized communication, or loyalty programs. Telecommunication companies, for example, leverage churn prediction models to identify customers likely to switch providers, allowing them to offer customized retention plans.
3. Personalized Marketing & Customer Experience: Data science fuels highly personalized marketing efforts. By analyzing individual customer data, businesses can tailor their marketing messages, product recommendations, and offers to resonate with each customer's unique preferences and needs. This leads to increased engagement, improved conversion rates, and stronger customer relationships. Netflix's recommendation engine is a prime example of personalized customer experience driven by data science.
4. Sales Forecasting: Accurate sales forecasting is crucial for effective business planning. Data science techniques such as time series analysis and regression modeling can analyze historical sales data, market trends, and economic indicators to generate more precise sales forecasts. This allows businesses to optimize resource allocation, inventory management, and sales team strategies. Automotive companies, for instance, use sales forecasting models to predict future demand, enabling optimized production planning.
5. Lead Scoring and Qualification: Data science can significantly improve lead scoring and qualification processes. By analyzing various data points such as website activity, email engagement, and social media interactions, algorithms can assess the likelihood of a lead converting into a customer. This helps sales teams prioritize high-potential leads and focus their efforts on the most promising prospects. SaaS companies, for example, often use lead scoring models to prioritize high-value prospects for their sales teams.
The Interplay of Big Data, Machine Learning, and AI
The increasing volume, velocity, and variety of customer data necessitate the use of big data technologies and machine learning (ML) algorithms. Big data platforms handle the massive datasets generated by modern CRM systems, while ML algorithms extract meaningful insights from this data. Artificial intelligence (AI) further enhances this process by automating tasks such as customer service interactions, personalized recommendations, and predictive modeling. The combination of these technologies significantly expands the capabilities of CRM, enabling more sophisticated analytics and automation. For example, AI-powered chatbots can handle routine customer inquiries, freeing up human agents to focus on more complex issues.
The Connection Between Data Privacy and Ethical Considerations
The effective utilization of data science in CRM requires a strong emphasis on data privacy and ethical considerations. Businesses must ensure compliance with relevant data protection regulations, such as GDPR and CCPA. Transparency and informed consent are paramount. Customers must be aware of how their data is being collected, used, and protected. Ethical considerations also include responsible use of AI and algorithms, avoiding bias and discrimination in decision-making processes. Building trust with customers through responsible data handling is critical for maintaining long-term relationships and avoiding reputational damage.
Connecting Data Security and CRM Data Science
Robust data security measures are crucial when integrating data science into CRM. This includes implementing strong access controls, encryption techniques, and regular security audits to protect sensitive customer data from unauthorized access and breaches. Data anonymization and pseudonymization techniques can further enhance privacy while still allowing for valuable data analysis. Investing in secure infrastructure and adhering to best practices in data security is essential for building and maintaining customer trust.
Deep Dive into Data Security in the Context of Data Science and CRM
Data security is paramount when leveraging data science within a CRM system. The potential for data breaches, misuse, or unauthorized access necessitates a comprehensive security strategy. Key factors include:
- Access Control: Implementing strict role-based access control (RBAC) ensures that only authorized personnel have access to sensitive data.
- Data Encryption: Encrypting data both in transit and at rest protects it from interception or unauthorized access.
- Regular Security Audits: Conducting regular security assessments and penetration testing identifies vulnerabilities and strengthens the system’s security posture.
- Intrusion Detection Systems (IDS): Implementing IDS monitors system activity for suspicious patterns and alerts administrators to potential threats.
- Data Loss Prevention (DLP): DLP tools prevent sensitive data from leaving the organization's network without authorization.
Common Questions (FAQ)
Q1: What are the main challenges of integrating data science into CRM?
A1: Challenges include data quality issues, the need for skilled data scientists, integration complexities, and ensuring data privacy and security.
Q2: How much does it cost to implement data science in a CRM?
A2: Costs vary greatly depending on the complexity of the implementation, the size of the dataset, and the level of expertise required.
Q3: What are some key performance indicators (KPIs) to measure the success of data science in CRM?
A3: Key KPIs include customer retention rate, customer lifetime value, conversion rates, sales growth, and customer satisfaction scores.
Q4: Can small businesses benefit from data science in CRM?
A4: Yes, even small businesses can benefit from simpler data science applications such as basic customer segmentation and churn prediction.
Q5: What are the ethical considerations related to using data science in CRM?
A5: Ethical considerations include data privacy, bias in algorithms, transparency, and responsible use of AI.
Q6: How can I ensure the data used for analysis is accurate and reliable?
A6: Data quality is crucial. Implementing data validation processes, data cleaning techniques, and regular data audits are vital for ensuring data accuracy and reliability.
Actionable Tips for Implementing Data Science in CRM
- Assess your data: Evaluate the quality, completeness, and relevance of your customer data.
- Define your objectives: Identify specific business goals you want to achieve with data science.
- Choose the right tools: Select appropriate data science tools and technologies that align with your needs.
- Build a skilled team: Invest in training your existing staff or hiring data scientists.
- Start with a pilot project: Begin with a smaller project to test the effectiveness of your approach.
- Monitor and iterate: Continuously monitor your results and adjust your strategies as needed.
- Prioritize data security and privacy: Implement robust security measures to protect sensitive data.
- Foster a data-driven culture: Encourage data-informed decision-making throughout your organization.
Conclusion
The synergy between data science and CRM offers unprecedented opportunities for businesses to understand their customers, optimize their operations, and drive significant growth. By leveraging the power of data science, organizations can move beyond traditional CRM capabilities and unlock the true potential of their customer data. The effective integration of data science, coupled with a robust data security framework and a commitment to ethical practices, will be a defining factor in business success in the years to come. The future of CRM is undoubtedly data-driven, promising a more personalized, efficient, and profitable customer experience. Embracing this transformation is not simply an option; it is a necessity for remaining competitive in today's dynamic marketplace.
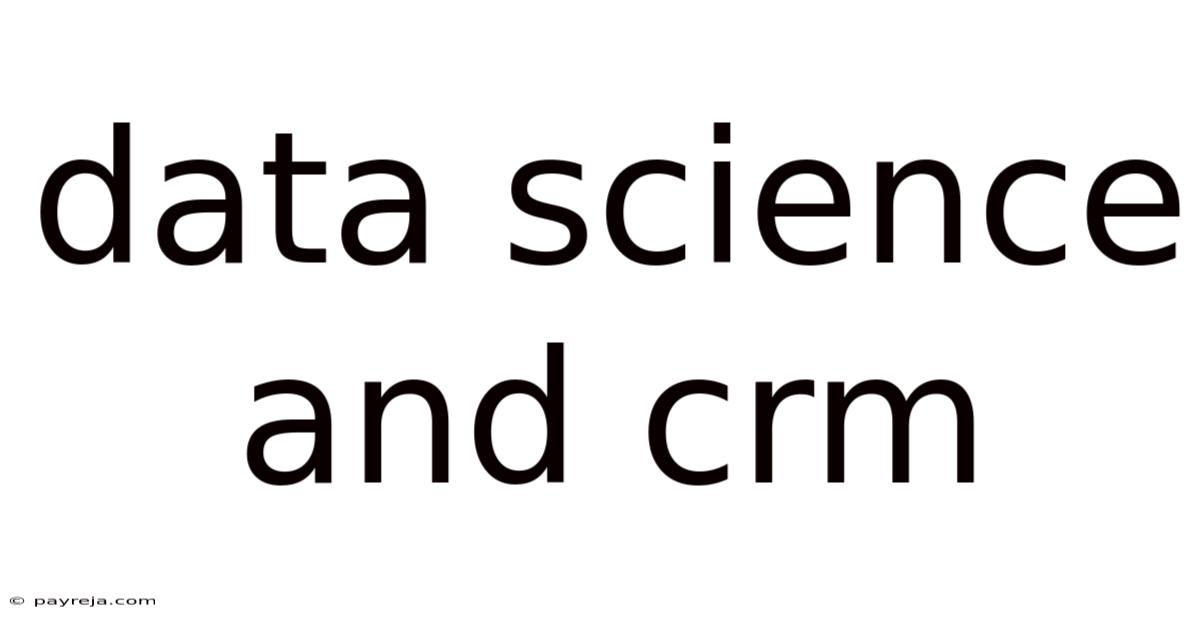
Thank you for visiting our website wich cover about Data Science And Crm. We hope the information provided has been useful to you. Feel free to contact us if you have any questions or need further assistance. See you next time and dont miss to bookmark.
Also read the following articles
Article Title | Date |
---|---|
What Does Crm Database Stand For | Apr 15, 2025 |
Gym Cmr | Apr 15, 2025 |
La Creme De Paris | Apr 15, 2025 |
Crm Developer Toolkit | Apr 15, 2025 |
Crm Aviation Europe Limited Maidenhead | Apr 15, 2025 |