Data Mining And Crm
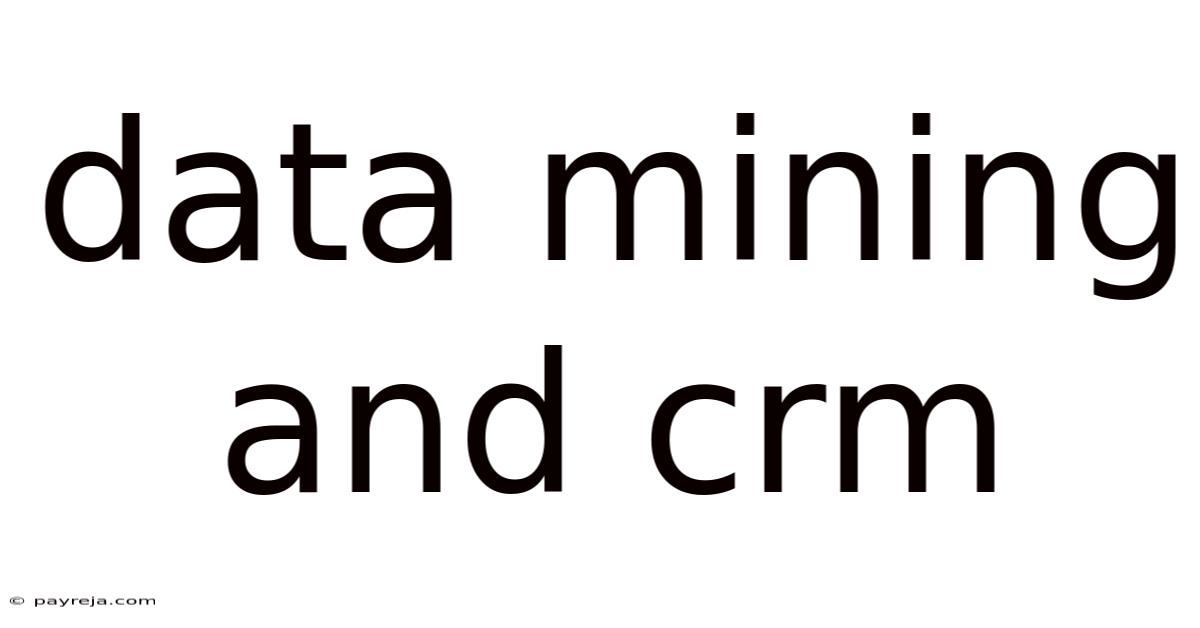
Discover more detailed and exciting information on our website. Click the link below to start your adventure: Visit Best Website meltwatermedia.ca. Don't miss out!
Table of Contents
Unlocking Customer Insights: The Power of Data Mining in CRM
What if harnessing the power of data mining could transform your CRM from a simple contact list into a strategic engine for growth? Data mining is no longer a futuristic concept; it's a critical tool for businesses seeking a competitive edge in today's data-driven world.
Editor’s Note: This article on data mining and CRM was published today, offering the latest insights and best practices in this rapidly evolving field.
Why Data Mining in CRM Matters
In today's hyper-competitive business landscape, understanding customers is paramount. Customer Relationship Management (CRM) systems are essential for managing interactions, tracking sales, and nurturing leads. However, a CRM brimming with data but lacking analytical depth is merely a digital filing cabinet. This is where data mining comes in. Data mining, a subset of data science, unearths hidden patterns, trends, and correlations within vast datasets residing in your CRM. This allows businesses to move beyond reactive customer service and into proactive, personalized engagement strategies. This leads to improved customer retention, increased sales conversion rates, and a deeper understanding of market dynamics. From predicting customer churn to identifying high-value prospects, data mining empowers businesses to make data-driven decisions, optimizing every stage of the customer lifecycle. The ability to segment customers based on precise characteristics, predict future behavior, and tailor marketing campaigns are key benefits that significantly contribute to business success and profitability.
Article Overview
This article explores the synergy between data mining and CRM, detailing how businesses can leverage this powerful combination to achieve strategic goals. It will cover key data mining techniques applicable to CRM data, the importance of data quality, ethical considerations, and practical strategies for implementing data mining within a CRM system. Readers will gain a clear understanding of how to extract actionable insights from their CRM data, leading to improved customer relationships and increased revenue generation.
Research Methodology and Data Sources
This article draws upon a comprehensive review of academic research papers published in reputable journals like the Journal of Marketing Research and MIS Quarterly. Industry reports from Gartner, Forrester, and IDC have also been consulted to provide current market trends and best practices. Case studies from various industry sectors (e.g., retail, finance, healthcare) illustrate the practical applications of data mining in CRM. The approach taken emphasizes a clear, structured presentation of information, using real-world examples and data-driven evidence to support key arguments.
Key Takeaways: Essential Insights from Data Mining in CRM
Insight | Description |
---|---|
Improved Customer Segmentation | Identify distinct customer groups based on demographics, behavior, and preferences for targeted marketing. |
Predictive Modeling for Churn Reduction | Forecast customer attrition risk and proactively intervene to retain valuable customers. |
Enhanced Lead Scoring & Qualification | Prioritize high-potential leads based on their likelihood to convert into paying customers. |
Personalized Customer Experience | Tailor interactions and offers to individual customer needs and preferences, enhancing engagement and loyalty. |
Optimized Marketing Campaign ROI | Measure campaign effectiveness and allocate resources efficiently based on data-driven insights. |
Improved Sales Forecasting & Planning | Predict future sales trends and adjust strategies accordingly to maximize revenue and profitability. |
Data Mining Techniques in CRM
Several data mining techniques are particularly useful when working with CRM data:
-
Association Rule Mining: This identifies relationships between different customer attributes and behaviors. For example, it might reveal that customers who purchased product A also tend to purchase product B, allowing for effective cross-selling and upselling strategies.
-
Classification: This technique categorizes customers into predefined groups (e.g., high-value, medium-value, low-value) based on their characteristics and past behavior. This allows for targeted marketing campaigns and personalized customer service.
-
Regression: This predicts a continuous variable (e.g., customer lifetime value, future sales) based on a set of predictor variables. This is invaluable for sales forecasting and resource allocation.
-
Clustering: This groups customers based on similarity, revealing hidden segments within your customer base. This is particularly useful for identifying underserved niches or creating more effective customer personas.
The Importance of Data Quality
The success of data mining heavily relies on the quality of the data. Garbage in, garbage out is a crucial principle here. Inaccurate, incomplete, or inconsistent data will lead to flawed insights and ineffective strategies. Therefore, data cleaning and preprocessing are crucial steps before employing data mining techniques. This includes:
- Data Cleansing: Handling missing values, removing duplicates, and correcting inconsistencies.
- Data Transformation: Converting data into a suitable format for analysis (e.g., normalization, standardization).
- Data Reduction: Selecting relevant variables and reducing dimensionality to improve efficiency and accuracy.
Ethical Considerations in Data Mining
Ethical considerations are paramount when utilizing customer data. Transparency, data privacy, and security must be prioritized. Compliance with regulations like GDPR and CCPA is critical. Businesses must be transparent with customers about how their data is used and ensure they have the option to opt-out or control their data. The ethical implications of using data mining for discriminatory or manipulative purposes must be carefully considered.
Connecting Point: Predictive Analytics and CRM Data Mining
Predictive analytics plays a crucial role in enhancing the effectiveness of data mining within CRM systems. By leveraging historical data and advanced algorithms, businesses can forecast future customer behaviors, anticipate potential problems, and proactively optimize their strategies.
Roles and Real-World Examples:
-
Customer Churn Prediction: Telecommunications companies utilize predictive models to identify customers at high risk of churn, allowing them to proactively offer retention incentives.
-
Sales Forecasting: Retailers employ predictive analytics to forecast demand, optimize inventory levels, and avoid stockouts or overstocking.
-
Targeted Marketing: Financial institutions use predictive models to identify individuals most likely to respond positively to specific financial products, increasing campaign efficiency.
Risks and Mitigations:
-
Data Bias: Biased data can lead to inaccurate predictions and unfair outcomes. Mitigation strategies include careful data selection and validation, as well as regular model auditing.
-
Model Complexity: Overly complex models can be difficult to interpret and may not generalize well to new data. Simpler, more interpretable models are often preferable.
-
Lack of Transparency: Lack of transparency in model development and deployment can erode trust and raise ethical concerns. Clear explanations of how models work and their limitations are essential.
Impact and Implications:
The combined power of predictive analytics and data mining allows businesses to move beyond reactive decision-making and towards proactive, data-driven strategies. This leads to improved customer satisfaction, increased efficiency, and enhanced profitability. The long-term implication is a more customer-centric and competitive business model.
Reinforcing the Connection in the Conclusion:
Predictive analytics significantly enhances the value of data mining within CRM systems. By anticipating future behaviors and trends, businesses can proactively optimize their customer interactions, improve their marketing strategies, and ultimately drive greater profitability. The integration of these two powerful analytical approaches is essential for organizations seeking to thrive in today's data-driven economy.
Diving Deeper into Predictive Analytics
Predictive analytics uses statistical techniques, machine learning algorithms, and data mining methods to forecast future outcomes based on historical data. Common algorithms include linear regression, logistic regression, decision trees, and neural networks.
Cause-and-Effect Analysis:
For example, an analysis might reveal a strong correlation between customer engagement on social media and subsequent purchases. This understanding allows businesses to tailor their social media strategies to increase customer engagement and drive sales.
Frequently Asked Questions (FAQ)
-
What is the difference between data mining and data analysis? Data analysis is a broader term encompassing various techniques to explore and interpret data. Data mining is a specific type of data analysis focusing on discovering hidden patterns and insights from large datasets.
-
What kind of CRM systems are best suited for data mining? CRMs with robust reporting and analytics capabilities, API access, and the ability to integrate with external data sources are ideal for data mining.
-
How much does data mining for CRM cost? The cost varies depending on the complexity of the project, the size of the dataset, and the expertise required. Costs can range from relatively low for basic analysis to substantial for large-scale projects involving advanced algorithms.
-
What skills are needed for data mining in CRM? Data mining requires expertise in statistics, machine learning, data visualization, and database management. Strong programming skills (e.g., Python, R) are also essential.
-
How long does it take to implement data mining in CRM? Implementation time depends on the project's scope and complexity. Simple projects can be implemented within weeks, while larger projects might take several months.
-
What are the potential risks of misinterpreting data mining results? Misinterpreting results can lead to flawed business decisions, wasted resources, and damage to customer relationships. Careful validation and interpretation are crucial.
Actionable Tips on Data Mining in CRM
-
Define Clear Objectives: Identify specific business goals you want to achieve through data mining (e.g., increase customer retention, improve lead conversion rates).
-
Ensure Data Quality: Cleanse and preprocess your data to ensure accuracy and consistency.
-
Select Appropriate Techniques: Choose data mining techniques that are relevant to your objectives and data characteristics.
-
Validate Results: Verify the accuracy and reliability of your findings using various methods.
-
Iterate and Refine: Data mining is an iterative process. Continuously monitor, refine, and adapt your models based on new data and insights.
-
Visualize Insights: Use dashboards and visualizations to present your findings clearly and effectively.
-
Implement Actions: Translate your insights into actionable strategies and tactics.
-
Monitor and Measure: Track the impact of your data mining efforts and make adjustments as needed.
Strong Final Conclusion
Data mining and CRM represent a powerful combination for businesses seeking to unlock the full potential of their customer data. By combining advanced analytical techniques with effective CRM strategies, organizations can gain a profound understanding of customer behavior, improve customer relationships, and drive significant business growth. The insights gained from data mining are not merely theoretical; they translate directly into actionable strategies that enhance customer experiences, optimize marketing campaigns, and increase profitability. Embracing data-driven decision-making is no longer optional; it's essential for survival and success in today’s competitive market.
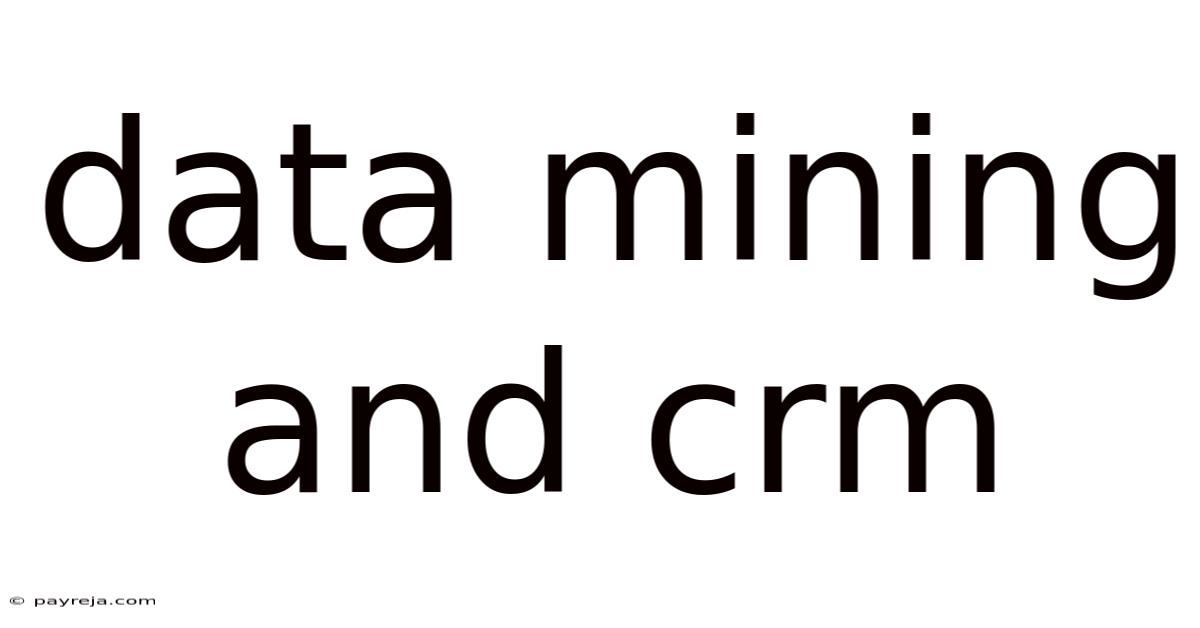
Thank you for visiting our website wich cover about Data Mining And Crm. We hope the information provided has been useful to you. Feel free to contact us if you have any questions or need further assistance. See you next time and dont miss to bookmark.
Also read the following articles
Article Title | Date |
---|---|
Moxie Crm Reddit | Apr 15, 2025 |
Pabau Crm | Apr 15, 2025 |
What Is Moxi Treatment | Apr 15, 2025 |
Mywellness App | Apr 15, 2025 |
Black Sheep Crm | Apr 15, 2025 |