Data Analysis And Crm
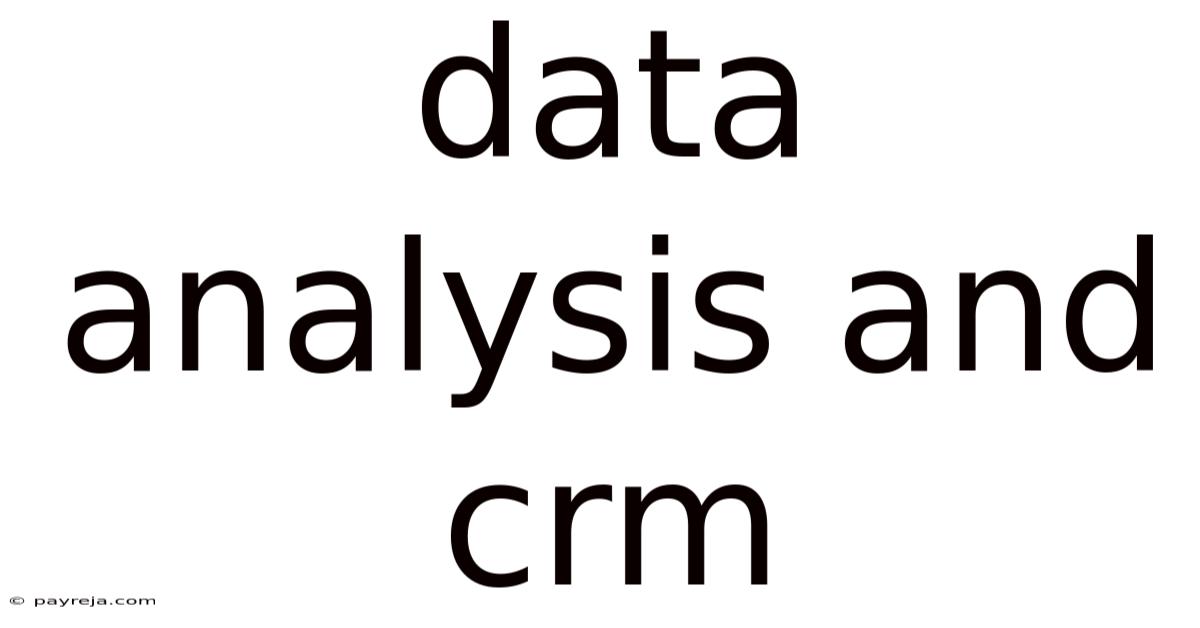
Discover more detailed and exciting information on our website. Click the link below to start your adventure: Visit Best Website meltwatermedia.ca. Don't miss out!
Table of Contents
Unlocking Growth: The Power of Data Analysis in CRM
What if harnessing the power of data analysis within your CRM could dramatically improve customer relationships and boost your bottom line? Data-driven CRM strategies are no longer a luxury; they are a necessity for thriving in today's competitive landscape.
Editor’s Note: This article on data analysis and CRM was published today, offering the latest insights and best practices in leveraging data for improved customer relationship management.
Why Data Analysis in CRM Matters
In today's business world, customer data is the new gold. A Customer Relationship Management (CRM) system acts as a central repository for this valuable information, encompassing everything from contact details and purchase history to customer service interactions and marketing campaign responses. However, the raw data within a CRM is just that – raw. Its true potential is unlocked through effective data analysis. This analysis transforms data into actionable insights, empowering businesses to personalize customer experiences, optimize marketing efforts, improve sales strategies, and ultimately, drive revenue growth. Understanding customer segmentation, predicting churn, and identifying high-value customers are all made possible through the insightful application of data analytics within the CRM framework. This directly impacts key performance indicators (KPIs) like customer lifetime value (CLTV), customer acquisition cost (CAC), and return on investment (ROI) across various business functions.
Article Overview
This article will delve into the crucial intersection of data analysis and CRM. It will cover key aspects of data analysis within a CRM context, exploring techniques, benefits, challenges, and best practices. Readers will learn how to leverage data for customer segmentation, predictive modeling, and personalized marketing, ultimately improving customer engagement and business outcomes. The article will also examine the relationship between data quality and analytical accuracy, highlighting the importance of data cleansing and validation. Finally, it will provide actionable strategies for implementing and maximizing the benefits of data-driven CRM.
Data Analysis Techniques in CRM
Several data analysis techniques prove invaluable when working with CRM data. These include:
-
Descriptive Analytics: This foundational level involves summarizing historical data to understand past performance. For example, analyzing past sales data to identify top-performing products or customer segments. Key metrics here include average order value (AOV), conversion rates, and customer churn rate.
-
Diagnostic Analytics: This goes a step further, exploring the reasons behind observed trends. Why did sales decline in a particular region? Diagnostic analytics uses techniques like drill-down analysis and data mining to uncover root causes.
-
Predictive Analytics: This leverages historical data and statistical modeling to forecast future outcomes. Predictive models can help identify customers at risk of churn, predict future sales, or optimize marketing campaign targeting. Machine learning algorithms play a vital role in this area.
-
Prescriptive Analytics: This is the most advanced form, using predictive analytics to recommend actions to optimize business outcomes. For example, a prescriptive model might suggest personalized offers to retain at-risk customers or optimize pricing strategies.
Data Quality: The Foundation of Effective Analysis
The accuracy and reliability of CRM data analysis directly depend on data quality. Inaccurate or incomplete data leads to flawed insights and ineffective decision-making. Therefore, data cleansing and validation are critical steps:
-
Data Cleansing: This involves identifying and correcting errors, inconsistencies, and redundancies in the data. This might include handling missing values, standardizing data formats, and removing duplicate entries.
-
Data Validation: This ensures the accuracy and completeness of the data. It involves verifying data against known sources and applying rules to identify inconsistencies.
Customer Segmentation: A Powerful Application
Customer segmentation is a cornerstone of effective CRM. By grouping customers based on shared characteristics (demographics, purchase behavior, engagement levels), businesses can tailor their marketing and sales efforts for maximum impact. Data analysis techniques, including clustering and classification algorithms, are used to identify optimal customer segments. For example, segmenting customers into high-value, medium-value, and low-value groups allows businesses to focus resources on acquiring and retaining the most profitable customers.
Predictive Modeling for Churn Reduction
Customer churn – the loss of customers – is a significant concern for many businesses. Predictive modeling can help identify customers at high risk of churning. By analyzing factors such as purchase frequency, engagement levels, and customer service interactions, businesses can build models that predict the likelihood of churn. This allows for proactive intervention, such as personalized offers or targeted communication, to retain these at-risk customers.
Personalized Marketing and Sales:
Data analysis enables highly personalized marketing and sales strategies. By analyzing individual customer preferences and behavior, businesses can create targeted campaigns and offers that resonate with specific customer segments. This can involve personalized email marketing, customized product recommendations, or tailored sales pitches. The result is increased customer engagement, higher conversion rates, and improved customer satisfaction.
Connecting Data Analysis and CRM Strategies
Effective use of data analysis requires integrating it seamlessly into the CRM strategy. This involves:
-
Defining clear objectives: What business questions does the data analysis aim to answer? This clarity guides the selection of appropriate analytical techniques and metrics.
-
Choosing the right tools: Selecting the right CRM system and analytical tools is crucial. Consider factors such as scalability, ease of use, and integration capabilities.
-
Building a data-driven culture: Encouraging data-driven decision-making throughout the organization is essential for maximizing the value of CRM data analysis.
The Role of Data Visualization
Data visualization is critical for effectively communicating insights from CRM data analysis. Dashboards and reports that present data in a clear, concise, and visually appealing way are essential for making data accessible and understandable to decision-makers across the organization. Key metrics should be highlighted, and trends should be easily identifiable.
Key Takeaways: Data Analysis and CRM Success
Insight | Description |
---|---|
Data quality is paramount | Accurate and complete data is essential for reliable insights. |
Segmentation drives personalized strategies | Grouping customers based on shared characteristics enables targeted marketing and sales efforts. |
Predictive modeling anticipates churn | Identifying at-risk customers allows for proactive interventions to improve customer retention. |
Data visualization enhances communication | Clear and concise data visualizations make insights accessible to all stakeholders. |
Integration is key | Seamless integration of data analysis into CRM strategies is crucial for maximizing the value of data analysis. |
Continuous improvement is vital | Regularly reviewing and refining analytical processes is essential for maintaining optimal performance. |
Exploring the Connection Between Customer Lifetime Value (CLTV) and Data Analysis in CRM
Customer Lifetime Value (CLTV) represents the total revenue a business expects to generate from a single customer over their entire relationship. Data analysis plays a crucial role in accurately predicting and maximizing CLTV. By analyzing purchase history, engagement levels, and other relevant data points, businesses can create more precise CLTV models. This enables them to prioritize high-value customers, tailor their strategies to enhance customer loyalty, and ultimately boost profitability.
-
Roles and Real-World Examples: Companies like Amazon utilize detailed customer purchase history and browsing behavior to predict future purchases, offering personalized recommendations and targeted promotions to increase CLTV.
-
Risks and Mitigations: Inaccurate data or flawed models can lead to underestimation or overestimation of CLTV, resulting in inefficient resource allocation. Regular model validation and data cleansing are essential mitigations.
-
Impact and Implications: Accurate CLTV prediction allows for better resource allocation, optimized customer acquisition strategies, and improved pricing models, leading to significant financial benefits.
Reinforcing the Connection in the Conclusion
The relationship between CLTV and data analysis in CRM is symbiotic. Accurate CLTV prediction relies on robust data analysis, while data-driven strategies, informed by CLTV insights, lead to increased customer loyalty and ultimately higher CLTV. Understanding this dynamic is crucial for sustainable business growth.
Diving Deeper into Customer Lifetime Value (CLTV)
CLTV calculation methods vary, but generally involve predicting future revenue based on historical customer behavior. Factors like average purchase value, purchase frequency, and customer lifespan are incorporated into the model. More sophisticated models incorporate predictive analytics to account for factors like customer churn and changes in buying patterns. Businesses can then use this information to segment their customers based on their projected CLTV, focusing resources on acquiring and retaining high-value customers.
Frequently Asked Questions (FAQ)
-
Q: What is the best CRM for data analysis? A: The "best" CRM depends on specific business needs and budget. Popular choices include Salesforce, HubSpot, and Microsoft Dynamics 365, each offering robust analytical capabilities.
-
Q: How can I improve the accuracy of my CRM data? A: Regularly cleanse and validate your data, implement data entry best practices, and use data integration tools to ensure consistency across systems.
-
Q: What are the biggest challenges in using data analysis in CRM? A: Data quality issues, lack of skilled personnel, and integrating data analysis into existing workflows are common challenges.
-
Q: How can I measure the success of my data-driven CRM strategy? A: Track key metrics like customer churn rate, customer acquisition cost, conversion rates, and customer lifetime value.
-
Q: What are some ethical considerations when using customer data for analysis? A: Ensure compliance with data privacy regulations (GDPR, CCPA, etc.) and be transparent with customers about data usage.
-
Q: How can I get started with data analysis in my CRM? A: Begin by identifying key business questions, selecting appropriate analytical tools, and focusing on a specific area for improvement, such as customer retention or targeted marketing.
Actionable Tips on Data Analysis and CRM
-
Prioritize Data Quality: Implement robust data cleansing and validation processes.
-
Define Clear Objectives: Establish specific, measurable, achievable, relevant, and time-bound (SMART) goals for data analysis.
-
Segment Your Customers: Utilize data analysis to identify and target key customer segments.
-
Build Predictive Models: Develop models to predict churn and optimize marketing campaigns.
-
Personalize Customer Interactions: Leverage insights to create personalized marketing and sales strategies.
-
Visualize Your Data: Use dashboards and reports to effectively communicate insights to stakeholders.
-
Continuously Monitor and Improve: Regularly review and refine your data analysis processes based on performance metrics.
-
Invest in Training: Ensure your team has the skills and knowledge to effectively utilize CRM data analysis.
Strong Final Conclusion
The power of data analysis within CRM is undeniable. By effectively leveraging customer data, businesses can gain profound insights into customer behavior, personalize interactions, optimize marketing efforts, and ultimately drive substantial business growth. This article has explored key techniques, challenges, and best practices, equipping readers with the knowledge and strategies to unlock the full potential of their CRM data. Embracing data-driven CRM is not simply a trend; it's a strategic imperative for success in today's competitive marketplace. The journey to maximizing the value of CRM data is ongoing, requiring continuous learning, adaptation, and a commitment to leveraging data for improved customer relationships and enhanced business outcomes.
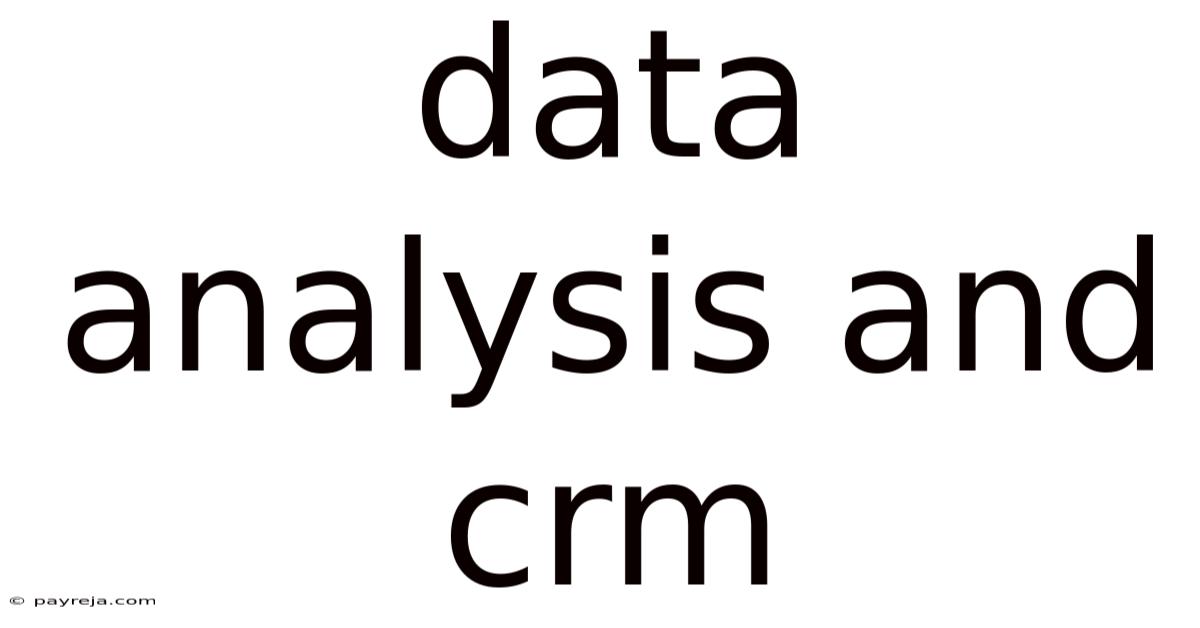
Thank you for visiting our website wich cover about Data Analysis And Crm. We hope the information provided has been useful to you. Feel free to contact us if you have any questions or need further assistance. See you next time and dont miss to bookmark.
Also read the following articles
Article Title | Date |
---|---|
Best Crm Marketing Automation | Apr 15, 2025 |
Crm Property Management Huntsville Al | Apr 15, 2025 |
Crm Assistant Jobs | Apr 15, 2025 |
Custom Cookie Ideas | Apr 15, 2025 |
Crm Services India Pvt Ltd Gurgaon | Apr 15, 2025 |