Crm Credit Risk Management
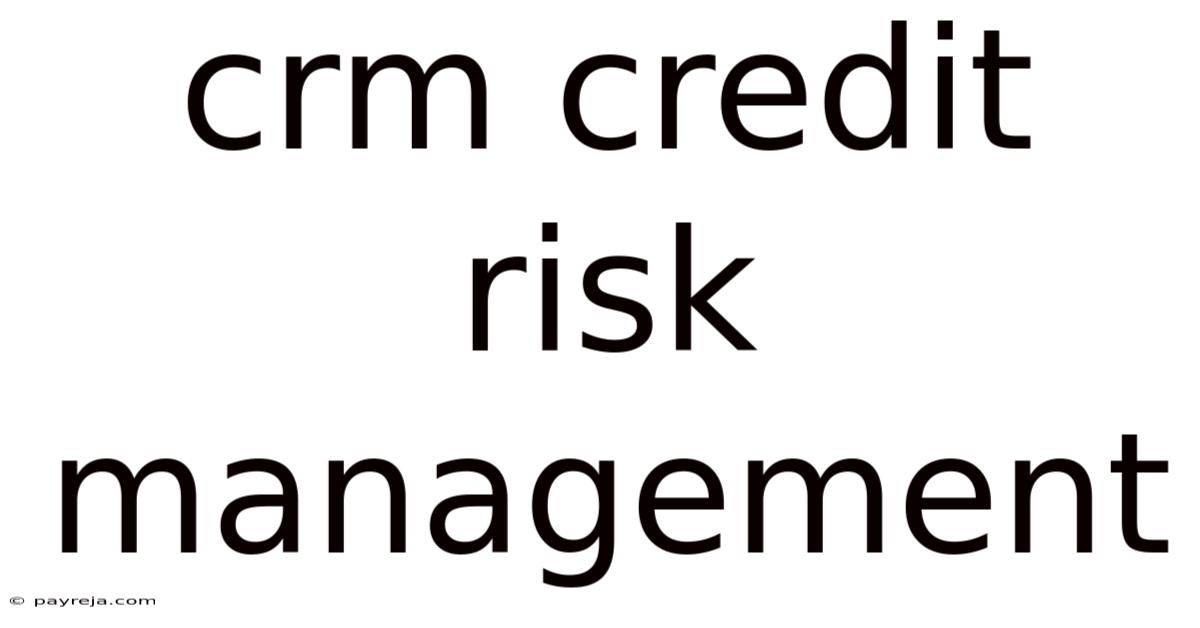
Discover more detailed and exciting information on our website. Click the link below to start your adventure: Visit Best Website meltwatermedia.ca. Don't miss out!
Table of Contents
Unlocking CRM's Power: Mastering Credit Risk Management
What if integrating CRM and credit risk management could dramatically reduce defaults and boost profitability? This powerful combination is transforming how businesses assess and manage risk, offering unprecedented levels of control and insight.
Editor’s Note: This article on CRM credit risk management was published today, offering the latest insights and best practices in this rapidly evolving field.
Why CRM Credit Risk Management Matters
Effective credit risk management is crucial for the financial health of any organization extending credit, from small businesses to large multinational corporations. Traditional methods often rely on isolated data silos, leading to inefficient processes, inaccurate risk assessments, and increased losses from defaults. Integrating Customer Relationship Management (CRM) systems with credit risk management processes offers a transformative solution. This integration allows businesses to leverage a holistic view of their customers, combining financial data with behavioral insights to create more accurate risk profiles and improve decision-making. This impacts profitability directly, reducing bad debt and optimizing lending strategies. The application spans diverse sectors, including banking, finance, telecommunications, and e-commerce, where extending credit is a core business function.
Article Overview
This article will explore the synergy between CRM and credit risk management, covering: the fundamental principles of effective credit risk management; how CRM data enhances risk assessment; practical applications across various industries; challenges and mitigation strategies; and actionable steps for implementing integrated CRM credit risk management. Readers will gain a comprehensive understanding of this powerful approach and learn how to leverage it for enhanced profitability and reduced risk.
The Foundations of Credit Risk Management
Credit risk management involves a systematic process for identifying, assessing, monitoring, and controlling the risk of borrowers defaulting on their financial obligations. This includes:
- Credit Scoring: Utilizing statistical models to assign a creditworthiness score based on various factors (credit history, income, debt-to-income ratio).
- Credit Application Processing: Efficiently handling applications, verifying information, and making lending decisions based on risk profiles.
- Monitoring and Collection: Tracking borrower behavior, identifying early warning signs of potential defaults, and implementing effective collection strategies.
- Regulatory Compliance: Adhering to relevant laws and regulations regarding lending and credit reporting.
How CRM Data Enhances Risk Assessment
CRM systems store a wealth of information about customers, far beyond financial data. Integrating this data with traditional credit scoring models significantly improves risk assessment. Here’s how:
- Behavioral Data: CRM captures interaction data, like purchasing habits, communication patterns, and website activity. These insights reveal much about a customer's financial responsibility and likelihood of repayment. For example, consistent late payments on non-credit accounts might indicate a higher risk of credit default.
- Demographic and Psychographic Data: CRM stores demographic information (age, location, occupation) and psychographic data (lifestyle, preferences). This helps build a more complete picture of the customer and identify potential risk factors. A customer's employment history, gleaned from their CRM profile, is highly relevant to their creditworthiness.
- Customer Service Interactions: Tracking interactions with customer service can reveal issues that might signal financial distress. Frequent inquiries about payment plans or hardship programs could indicate an increased risk of default.
Practical Applications Across Industries
The integration of CRM and credit risk management offers tangible benefits across numerous sectors:
- Banking and Finance: Banks use this integration to refine their loan approval process, personalize credit offers, and proactively identify at-risk borrowers.
- Telecommunications: Telecom companies leverage CRM data to manage postpaid accounts, predict churn (customers switching providers), and optimize collection strategies.
- E-commerce: Online retailers utilize CRM data to offer buy-now-pay-later options, assessing risk and managing potential losses efficiently.
- Retail: Retail stores offering store credit cards can use this integration to manage their credit risk effectively and target high-value customers with personalized offers.
Challenges and Mitigation Strategies
Implementing an integrated CRM credit risk management system presents several challenges:
- Data Integration Complexity: Combining data from diverse sources requires careful planning and potentially significant IT investment.
- Data Security and Privacy: Protecting sensitive customer data is paramount; robust security measures are essential.
- Model Accuracy and Bias: Credit scoring models must be regularly reviewed and updated to ensure accuracy and avoid bias.
- Cost and Return on Investment (ROI): Organizations need to carefully assess the costs involved against the potential benefits.
Mitigation Strategies:
- Phased Implementation: Start with a pilot project to test the integration before full-scale deployment.
- Data Governance and Security: Establish clear data governance policies and implement robust security measures.
- Model Validation and Monitoring: Regularly validate and monitor credit scoring models to ensure accuracy and fairness.
- Clear ROI Measurement: Define key performance indicators (KPIs) to measure the impact of the system on key metrics such as default rates and profitability.
Key Takeaways: Insights for Improved Risk Management
Insight | Description |
---|---|
Enhanced Risk Assessment | CRM data significantly improves accuracy in evaluating creditworthiness. |
Proactive Risk Management | Early identification of at-risk borrowers allows for timely intervention, minimizing losses. |
Improved Customer Experience | Personalized credit offers and efficient processes improve customer satisfaction. |
Increased Profitability | Reduced default rates and optimized lending strategies directly boost profitability. |
Regulatory Compliance | Integrated systems help ensure compliance with relevant regulations. |
Exploring the Connection Between Data Analytics and CRM Credit Risk Management
Data analytics plays a pivotal role in maximizing the effectiveness of CRM credit risk management. Sophisticated algorithms analyze CRM data, identifying patterns and trends that predict default risk more accurately than traditional methods. For example, machine learning models can analyze thousands of data points to identify subtle indicators of potential defaults that might be overlooked by human analysts.
Roles and Real-World Examples:
Data scientists build and maintain predictive models; risk managers interpret the outputs of these models and adjust lending strategies accordingly; IT professionals integrate data sources and ensure the system's functionality. A major bank might use data analytics to segment its customer base into risk tiers, tailoring credit offers and collection strategies to each segment.
Risks and Mitigations:
Overreliance on predictive models without human oversight can lead to biased decisions or inaccurate risk assessments. Regular model validation, rigorous data quality checks, and human-in-the-loop approaches are crucial mitigations.
Impact and Implications:
Data analytics enables personalized risk management, offering tailored credit terms and collection approaches based on individual customer profiles. This improves customer satisfaction while simultaneously reducing default rates and enhancing profitability.
Reinforcing the Connection in the Conclusion:
Data analytics is not merely an add-on; it's the engine that drives effective CRM credit risk management. By leveraging the power of data analytics, organizations can move beyond basic credit scoring, gaining a deeper understanding of customer behavior and proactively managing risk.
Diving Deeper into Data Analytics
Data analytics involves a range of techniques, from descriptive statistics (summarizing past data) to predictive modeling (forecasting future outcomes). Advanced techniques such as machine learning and deep learning offer even greater predictive power. These models can identify non-linear relationships between variables, uncovering subtle indicators of risk that might be missed by simpler methods. For example, a machine learning model might identify a correlation between a customer's social media activity and their likelihood of defaulting on a loan.
Cause-and-Effect Analysis:
Increased data availability and computational power have led to the development of more sophisticated predictive models, which in turn have resulted in more accurate risk assessments and improved profitability for financial institutions.
Frequently Asked Questions (FAQ)
Q1: What is the cost of implementing a CRM credit risk management system?
A1: The cost varies depending on the size of the organization, the complexity of the system, and the level of customization required. It involves initial investment in software, hardware, integration services, and ongoing maintenance costs.
Q2: How long does it take to implement such a system?
A2: Implementation time varies widely depending on the complexity of the project. It can range from several months to more than a year.
Q3: What are the key performance indicators (KPIs) for measuring the success of this system?
A3: Key KPIs include: default rate, collection efficiency, return on investment (ROI), customer satisfaction, and regulatory compliance.
Q4: How can we ensure the accuracy and fairness of credit scoring models?
A4: Regular model validation, rigorous data quality checks, and ongoing monitoring are essential to ensure both accuracy and fairness. Explainable AI (XAI) techniques can help understand model decision-making, mitigating bias.
Q5: What are the security risks associated with integrating CRM and credit risk management?
A5: Key security risks include unauthorized access to sensitive customer data and data breaches. Robust security measures, including encryption, access controls, and regular security audits, are essential.
Q6: How can we address concerns about customer privacy?
A6: Compliance with relevant data privacy regulations (like GDPR) is paramount. Transparency with customers about data usage and obtaining explicit consent are crucial aspects.
Actionable Tips on CRM Credit Risk Management
- Assess Current Processes: Thoroughly analyze existing credit risk management procedures to identify areas for improvement.
- Data Integration Strategy: Develop a comprehensive plan for integrating data from various sources, ensuring data quality and consistency.
- Model Selection and Validation: Choose appropriate credit scoring models and validate their accuracy and fairness regularly.
- Security and Privacy Measures: Implement robust security measures to protect sensitive customer data and comply with relevant regulations.
- Monitoring and Reporting: Establish a system for monitoring key performance indicators (KPIs) and generating regular reports.
- Continuous Improvement: Regularly evaluate the system's performance and make necessary adjustments to optimize efficiency and effectiveness.
- Employee Training: Train employees on using the system and interpreting the results of credit risk assessments.
- Customer Communication: Communicate clearly with customers about credit policies and procedures.
Strong Final Conclusion
The integration of CRM and credit risk management, powered by data analytics, represents a significant advancement in managing financial risk. By leveraging the rich data within CRM systems and applying sophisticated analytical techniques, organizations can significantly reduce defaults, improve profitability, and enhance customer relationships. This article has explored the fundamental principles, practical applications, and challenges involved. By implementing the actionable tips provided, organizations can effectively harness the power of this approach, securing a more robust and profitable future. The ongoing evolution of data analytics and machine learning promises to further refine this powerful combination, unlocking even greater opportunities for risk mitigation and business growth.
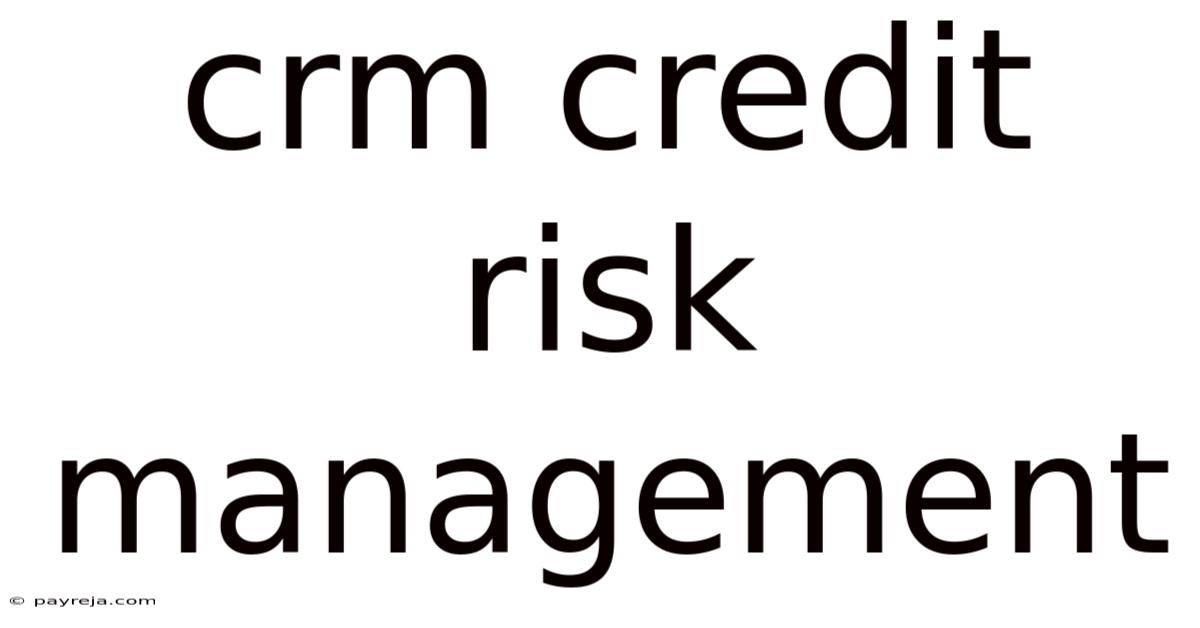
Thank you for visiting our website wich cover about Crm Credit Risk Management. We hope the information provided has been useful to you. Feel free to contact us if you have any questions or need further assistance. See you next time and dont miss to bookmark.
Also read the following articles
Article Title | Date |
---|---|
Best Crm For Independent Insurance Agents | Apr 08, 2025 |
Cms Crm System | Apr 08, 2025 |
Best 2015 | Apr 08, 2025 |
Top 5 Advertising Agencies | Apr 08, 2025 |
Top 10 Freight Brokers | Apr 08, 2025 |