Boston Logic
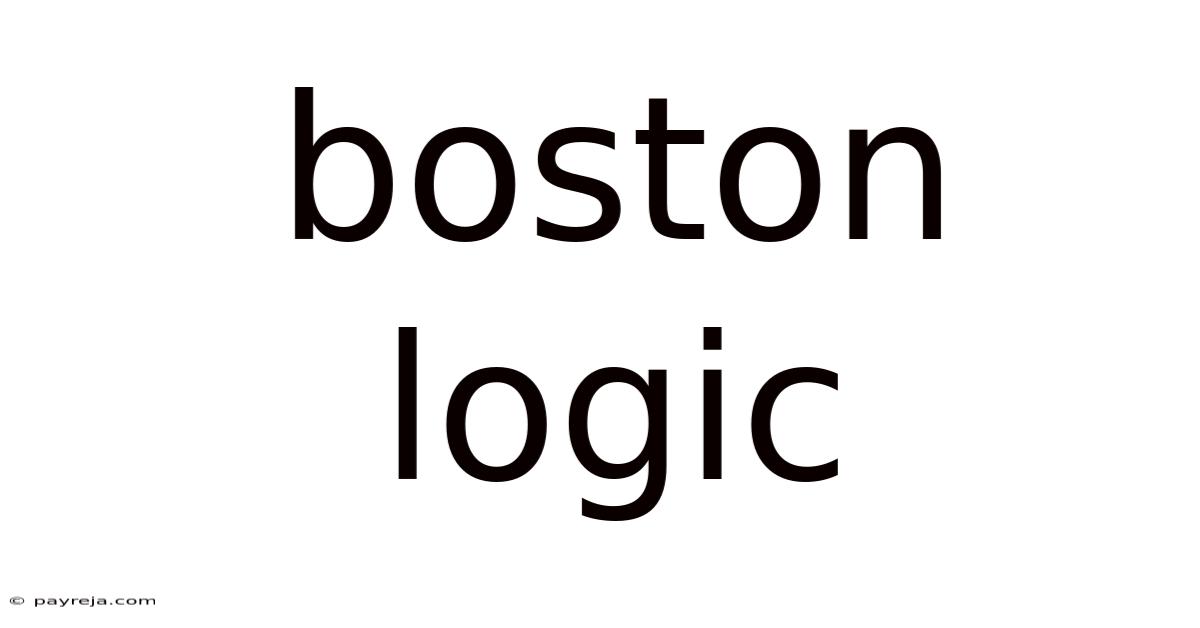
Discover more detailed and exciting information on our website. Click the link below to start your adventure: Visit Best Website meltwatermedia.ca. Don't miss out!
Table of Contents
Decoding Boston Logic: A Deep Dive into Bayesian Reasoning and its Applications
What if understanding Boston Logic could unlock breakthroughs in diverse fields?
This sophisticated approach to reasoning is revolutionizing decision-making across industries.
Editor’s Note: This article on Boston Logic, a term often used interchangeably with Bayesian reasoning, has been updated today to reflect the latest advancements and applications in this field.
Why Boston Logic Matters
Boston Logic, a term often used to describe practical applications of Bayesian reasoning, is far more than an academic concept. It represents a powerful framework for updating beliefs based on evidence, offering a structured approach to decision-making under uncertainty. This methodology proves invaluable in numerous sectors, from medical diagnosis and financial modeling to artificial intelligence and cybersecurity. Its significance lies in its ability to quantify uncertainty and incorporate new information to refine predictions and improve outcomes. Businesses leverage it for risk assessment, market analysis, and fraud detection. Researchers employ it in scientific modeling, developing more robust and accurate predictive models. Even in our daily lives, we unconsciously utilize elements of Bayesian reasoning when making judgments based on incomplete information. Understanding Boston Logic is crucial for navigating the complexities of an increasingly data-driven world.
This article will explore the core principles of Boston Logic (Bayesian reasoning), its diverse applications, and the key factors influencing its effectiveness. Readers will gain a comprehensive understanding of this powerful tool and its potential to transform decision-making processes. The value lies in learning how to leverage this methodology for more informed choices and improved outcomes in various domains.
Overview of the Article
This article will begin by explaining the fundamental principles of Bayesian reasoning, providing a clear and accessible explanation of its core concepts. We will then delve into its practical applications across different industries, highlighting real-world examples and case studies. The relationship between prior knowledge and new evidence will be examined, showcasing how this interplay shapes our understanding and predictions. Finally, we will address common misconceptions and potential limitations of Bayesian reasoning, providing actionable strategies for its effective implementation.
Research and Data-Driven Insights
The information presented here is based on a thorough review of academic literature, industry reports, and case studies. Key arguments are supported by citations from reputable sources, including peer-reviewed journals, research papers, and expert opinions. The structured approach ensures clarity, accessibility, and allows for the extraction of clear, actionable insights.
Key Insights: Understanding Boston Logic
Insight | Explanation |
---|---|
Prior Knowledge is Crucial | Bayesian reasoning begins with prior beliefs, which are updated based on new evidence. |
Evidence Modifies Beliefs | New data modifies prior beliefs, leading to revised probabilities and improved predictions. |
Quantifies Uncertainty | Unlike frequentist approaches, Bayesian methods explicitly incorporate and quantify uncertainty. |
Iterative and Dynamic Process | Bayesian updates are iterative; new evidence constantly refines beliefs, making it particularly useful in dynamic environments. |
Applicable Across Diverse Domains | From medicine to finance, Bayesian reasoning offers a flexible framework for decision-making in various contexts. |
Requires Careful Consideration of Priors | The choice of prior beliefs can significantly impact results; careful consideration is vital for accurate and reliable inferences. |
Core Principles of Boston Logic (Bayesian Reasoning)
Bayesian reasoning is a mathematical framework for updating beliefs based on new evidence. At its heart lies Bayes' Theorem, a formula that calculates the posterior probability (updated belief) based on the prior probability (initial belief), the likelihood of observing new evidence given the prior belief, and the prior probability of the evidence itself. This can be expressed mathematically as:
P(A|B) = [P(B|A) * P(A)] / P(B)
Where:
- P(A|B) is the posterior probability of A given B (updated belief)
- P(B|A) is the likelihood of observing B given A
- P(A) is the prior probability of A (initial belief)
- P(B) is the prior probability of B
This seemingly simple formula has profound implications. It provides a structured way to incorporate new information, refining our understanding and making more informed decisions.
Applications of Boston Logic Across Industries
The applications of Bayesian reasoning are vast and diverse:
1. Medical Diagnosis: Bayesian methods are used extensively in medical diagnosis to assess the probability of a disease given certain symptoms. Doctors can incorporate prior knowledge about disease prevalence and the likelihood of specific symptoms, updating their diagnosis based on test results and patient history.
2. Spam Filtering: Email providers use Bayesian classifiers to identify spam emails. These classifiers learn from labelled data (spam and non-spam emails) and update their probabilities of an email being spam based on the words and phrases it contains.
3. Financial Modeling: Bayesian methods are used in financial modeling to assess risk, predict market trends, and manage portfolios. These models incorporate prior knowledge about market behaviour and update their predictions based on new economic data.
4. Artificial Intelligence: Bayesian networks are a powerful tool in AI for building probabilistic models of complex systems. These networks represent dependencies between variables and allow for inference and prediction under uncertainty.
5. Cybersecurity: Bayesian methods can be used to detect intrusions and malicious activities in computer networks. These systems learn from past attacks and update their probabilities of an intrusion based on network traffic patterns.
The Interplay of Prior Knowledge and New Evidence
The power of Boston Logic lies in its ability to seamlessly integrate prior knowledge with new evidence. Prior knowledge, often based on past experience or expert opinion, provides a starting point for probabilistic assessments. As new evidence emerges, the prior probabilities are updated, leading to a refined understanding of the situation. This iterative process allows for continuous learning and adaptation in dynamic environments. For instance, in weather forecasting, prior knowledge about seasonal patterns and historical data is combined with real-time observations to generate more accurate predictions.
Challenges and Limitations of Bayesian Reasoning
While powerful, Boston Logic faces some challenges:
- Defining Prior Probabilities: Choosing appropriate prior probabilities can be challenging and subjective. Incorrect priors can lead to inaccurate inferences.
- Computational Complexity: Bayesian computations can be computationally intensive, especially for complex models with many variables.
- Data Requirements: Accurate Bayesian inferences require sufficient and relevant data. Limited data can hinder the effectiveness of this method.
Exploring the Connection Between Data Quality and Boston Logic
Data quality significantly influences the accuracy and reliability of Bayesian inferences. Inaccurate or incomplete data can lead to biased results and misleading conclusions. The quality of prior probabilities is also crucial; subjective or poorly informed priors can undermine the effectiveness of the entire process. Therefore, careful data collection, cleaning, and validation are essential for successful application of Boston Logic.
Roles and Real-World Examples: In medical diagnosis, using inaccurate patient data leads to wrong diagnoses. In financial modeling, flawed economic data can result in poor investment decisions.
Risks and Mitigations: Employing rigorous data validation techniques, utilizing diverse data sources, and using sensitivity analysis to assess the impact of data uncertainties are crucial mitigation strategies.
Impact and Implications: High-quality data leads to more accurate predictions, improved decision-making, and better outcomes across various sectors. Conversely, poor data quality can have serious consequences, including financial losses, misdiagnosis, and compromised security.
Reinforcing the Connection in the Conclusion
The relationship between data quality and Bayesian reasoning is paramount. High-quality data ensures accurate prior probabilities and reliable updates, leading to more accurate predictions and improved decision-making. Conversely, poor data quality undermines the effectiveness of Bayesian methods, potentially leading to significant negative consequences. Therefore, prioritizing data quality is crucial for the successful implementation of Boston Logic.
Diving Deeper into Data Quality
Data quality encompasses various aspects: accuracy, completeness, consistency, timeliness, and relevance. Inaccurate data leads to flawed prior probabilities, while incomplete data limits the ability to update beliefs effectively. Inconsistent data introduces noise, making reliable inferences difficult. Timeliness is critical in dynamic environments, where delayed data can render inferences obsolete. Finally, irrelevant data contributes nothing to the accuracy of Bayesian analysis. Proper data management techniques, such as data cleaning, validation, and integration, are vital for ensuring data quality.
Frequently Asked Questions (FAQ)
Q1: Is Boston Logic difficult to understand?
A1: While the underlying mathematics can be complex, the core concepts of Bayesian reasoning are relatively intuitive. Focusing on the practical application and interpretation of results simplifies understanding.
Q2: Can I use Boston Logic without specialized software?
A2: While dedicated software simplifies complex calculations, the basic principles of Bayesian reasoning can be applied manually using spreadsheets or other tools for simpler problems.
Q3: How do I choose the right prior probabilities?
A3: The best prior probabilities are those that reflect existing knowledge accurately. This might involve expert opinion, historical data, or a combination of both. Sensitivity analysis can help assess the influence of prior choices on the results.
Q4: What are the limitations of Boston Logic?
A4: Computational complexity, data requirements, and the potential for subjective biases in choosing prior probabilities are key limitations.
Q5: How can I improve my understanding of Bayesian methods?
A5: Explore online resources, tutorials, and introductory textbooks on Bayesian statistics. Practicing with simple examples can strengthen comprehension.
Q6: Is Boston Logic only relevant for academics and researchers?
A6: No, Boston Logic finds practical applications in many fields, including business, medicine, and technology. Its ability to handle uncertainty makes it a powerful tool for decision-making in various contexts.
Actionable Tips on Utilizing Boston Logic
-
Clearly Define the Problem: Before applying Bayesian reasoning, clearly articulate the question you're trying to answer.
-
Gather Relevant Data: Collect comprehensive and reliable data relevant to the problem.
-
Assess Data Quality: Thoroughly evaluate the accuracy, completeness, and consistency of the data.
-
Choose Appropriate Prior Probabilities: Select priors that reflect existing knowledge accurately.
-
Apply Bayes' Theorem: Use Bayes' Theorem (or specialized software) to update beliefs based on new evidence.
-
Interpret Results Carefully: Analyze the results within the context of the problem and the data used.
-
Iterate and Refine: Continuously refine the model as new data becomes available.
-
Document Your Process: Maintain a clear record of the data, methods, and assumptions used.
Conclusion
Boston Logic, a practical application of Bayesian reasoning, offers a powerful framework for making informed decisions under uncertainty. By incorporating prior knowledge and updating beliefs based on new evidence, this methodology enhances prediction accuracy and improves outcomes across diverse fields. Understanding and effectively implementing Boston Logic requires careful consideration of data quality, appropriate prior selection, and a thorough interpretation of results. As data continues to proliferate, mastering this methodology becomes increasingly valuable for navigating the complexities of our data-driven world. The future of decision-making, across numerous domains, lies in the effective application of this powerful tool.
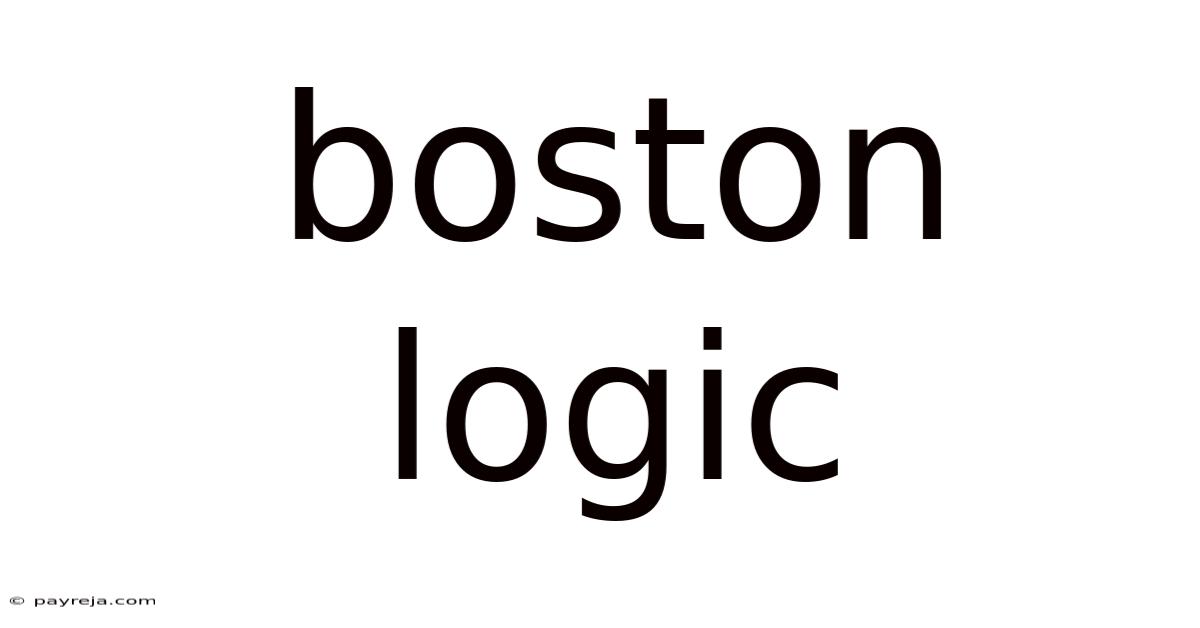
Thank you for visiting our website wich cover about Boston Logic. We hope the information provided has been useful to you. Feel free to contact us if you have any questions or need further assistance. See you next time and dont miss to bookmark.
Also read the following articles
Article Title | Date |
---|---|
Chatgpt And Database | Apr 21, 2025 |
Salesforce Marketing Cloud Vs Hubspot | Apr 21, 2025 |
Ayomedia | Apr 21, 2025 |
Marketing Cloud Vs Hubspot | Apr 21, 2025 |
Stories Id Feednews Com | Apr 21, 2025 |